For most of engineering history, optimization meant choosing what to lose. Do you want it cheaper, faster, or better? Pick two. Trade-offs were not signs of failure—they were the essence of the craft. Engineers didn't chase perfection; they balanced imperfection with grace. It was an art form built on scarcity: of time, of budget, of compute. But generative AI has changed the canvas.
Where engineers once tiptoed through a minefield of constraints, they now conduct symphonies of possibility. Instead of asking "What must we give up?", the new question is: How much more can we bring together?
Let's walk through three tectonic shifts reshaping the logic of engineering optimization.
Trend 1: Multidimensional Optimization
"Generative AI doesn't just find better answers—it redefines the questions, dissolving trade-offs and transforming optimization from a game of compromise into a symphony of possibility."
Traditional optimization felt like solving a Rubik's Cube with your eyes closed—improve one face, and the others scrambled. Engineers could fine-tune for speed or strength or sustainability, but never all three. Even the most advanced metaheuristics relied on simplifying assumptions, reducing complexity to make it computable.
Generative AI flips that constraint. It doesn't simplify the system—it dives headfirst into its complexity, embracing high-dimensional interactions and searching through vast combinatorial landscapes at breathtaking speed.
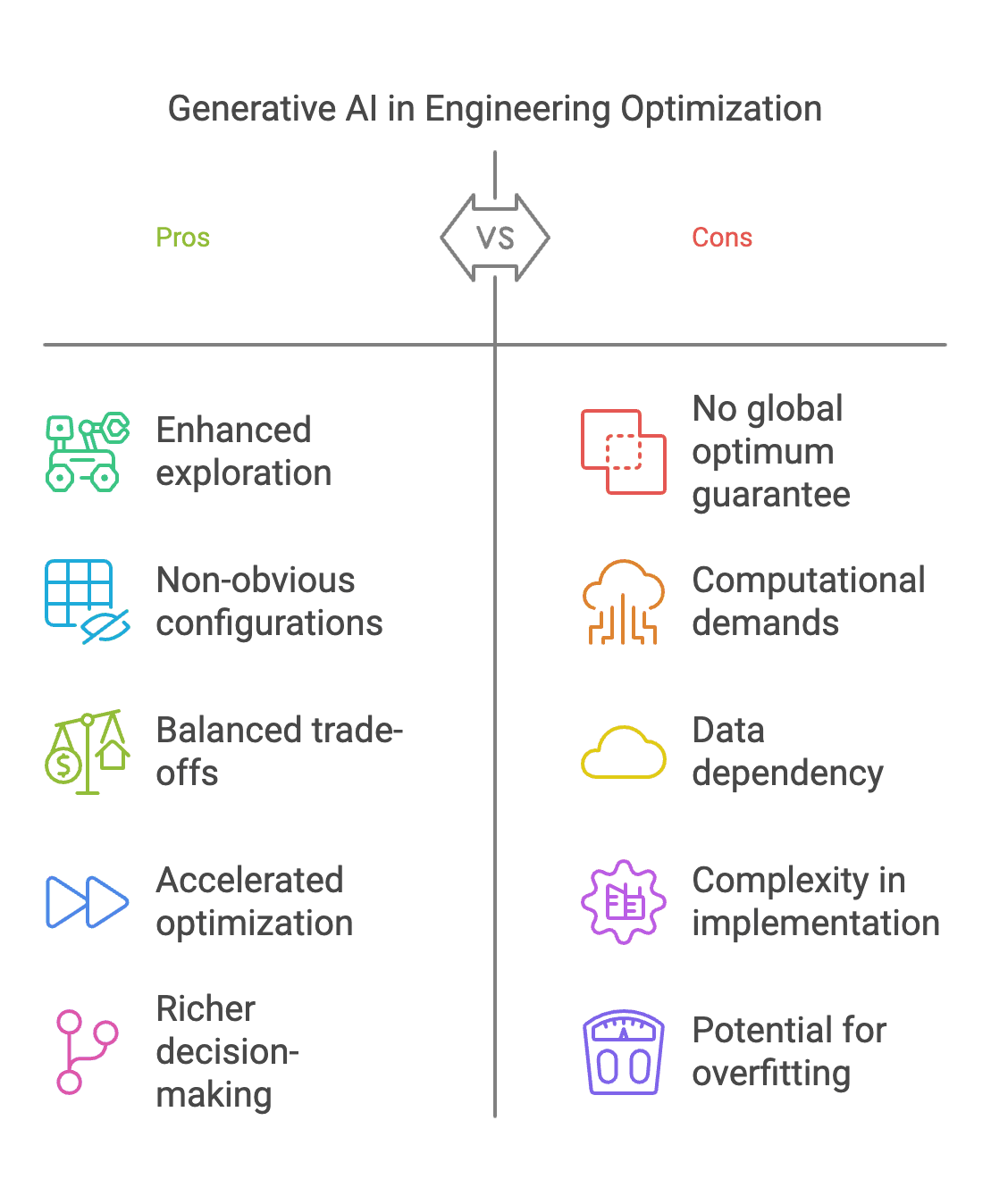
Figure 1: The Trade-offs of Generative AI in Engineering Optimization
Generative AI enhances engineering by enabling faster optimization, richer decision-making, and novel solutions, but it also introduces challenges like computational demands, complexity, and overfitting risks.
(Click image to view full size)
Take aerospace. Airbus used generative algorithms to reimagine internal aircraft partitions. The result? A lattice structure that mimicked bone growth—45% lighter yet equally strong. Fuel consumption dropped. CO₂ emissions fell. No trade-off—just transcendence.
Or consider the automotive industry. General Motors applied generative design to a seat bracket, once built from eight separate components. AI generated a single, elegant form that was 40% lighter and 20% stronger, cutting assembly time and waste simultaneously.
And the innovation doesn't stop with vehicle bodies. At NASA, engineers turned to generative design and additive manufacturing to reinvent heat exchangers for spaceflight. Inspired by natural patterns like bone structures and minimal surfaces, these components became up to 42% lighter and 30% more efficient. Using ultrasonic additive manufacturing, NASA created single-piece exchangers with no weak points, delivering better thermal performance in smaller, more reliable packages—vital when every gram matters in orbit.
This isn't just better engineering—it's more holistic engineering. Constraints become coordinates in a design space too large for the human mind but perfectly suited to machine exploration. And suddenly, the game shifts from choosing sacrifices to orchestrating synergies.
Trend 2: Real-Time Decision-Making with AI – From Reactive Design to Anticipatory Systems
"Generative AI turns crisis management into foresight, transforming reaction into resilience and ensuring problems are solved before they even surface."
In the past, engineering responded. Something failed, so you fixed it. Demand spiked, so you added capacity. Systems were designed around averages and reacted to outliers. But in a world moving at the speed of data, reactivity is simply too slow.
Enter generative AI, which transforms engineering from a discipline of response to one of foresight. Real-time inputs become living variables, not static assumptions. AI doesn't just process data—it predicts outcomes and preemptively adapts.
Take Israel's Iron Dome. Powered by AI-driven radar and machine learning, it analyzes incoming projectiles in milliseconds, calculates trajectory, and decides—on the fly—whether to intercept. Rockets bound for open fields are ignored. Threats to civilians are neutralized with pinpoint accuracy. In a single battery, AI orchestrates hundreds of simultaneous trajectories with over 90% effectiveness. It's not just defense—it's real-time choreography in a dynamic, high-stakes theater.
Or Bosch's AIoT factories. In Dresden and Hildesheim, embedded sensors stream real-time data from machines into predictive models. Generative AI creates synthetic defect images to train systems faster. The result? Downtime dropped by 30%, throughput rose, and maintenance costs fell—before a single part failed. These aren't smart factories. They're factories that think ahead.
Even in finance, reinforcement learning has redefined agility. AI agents simulate thousands of trading strategies per second, adapting in real time to market volatility. During the 2024 energy crisis, some models reduced portfolio drawdowns by 35% compared to human traders—because they didn't just model the economy. They learned from it, adjusted to it, and stayed ahead of it.
Engineering is no longer slow thinking applied to fast systems. With generative AI, it becomes fast intuition applied at scale—a system that sees the storm before it hits, and reroutes itself accordingly.
Trend 3: Accelerating Discovery and Innovation – Collapsing the Iteration Cycle
"Generative AI doesn't just speed up innovation—it reframes it, turning the process from a slow grind into a quantum leap, where the limits of human imagination and possibility are pushed further with every iteration."
Historically, innovation in engineering moved at the pace of physical trial and error. Design, prototype, test, revise—repeat. The process worked, but it was slow, expensive, and brittle. Every new idea demanded time and capital. Creativity had to be rationed. With generative AI, the bottleneck explodes.
AI doesn't just optimize what exists—it explores what could exist. It generates thousands of feasible alternatives in minutes, then simulates, scores, and refines them automatically. The loop from concept to insight compresses dramatically.
Consider circuit design. At MIT, the L2DC project trained reinforcement models to generate power-optimized chip layouts. The AI matched or exceeded human expert performance in a fraction of the time, while cutting energy usage by up to 80%—a leap crucial for IoT and edge computing.
In biomedical engineering, prosthetic limbs—once requiring months of bespoke fitting—are now co-designed by AI in hours. Digital twins simulate gait, stress, and balance for each individual patient, optimizing not just shape, but how the body adapts over time. Companies like Össur and Open Bionics report turnaround times slashed by 90%, and patient satisfaction soaring.
And even in quantum physics, where complexity reaches cosmic levels, AI is pushing frontiers. Researchers at the Flatiron Institute used neural networks to simplify quantum many-body problems once thought intractable—reducing equations from thousands to just a few dozen, without loss of accuracy.
The result? A new tempo of engineering. Ideas evolve in real time, iteration becomes exploration, and the question shifts from "What can we build?" to "How fast can we invent?"
Conclusion
Taken together, these trends mark the end of optimization as we knew it.
Where we once saw trade-offs, we now see harmony.
Where we once reacted, we now anticipate.
Where we once labored through cycles, we now iterate at the speed of thought.
But such power demands precision—and perspective. Optimization at this scale risks runaway complexity, opaque decision chains, or sustainability blind spots. That's why the engineer's role is evolving—from technician to orchestrator, from solver to steward. Because if AI can model infinite possibilities, it's up to us to choose which ones we bring into the world.
The next trail explores this orchestration not in theory, but in virtual reality—where digital twins become our most dynamic tools for managing complexity, predicting resilience, and designing systems that think as they evolve.
🌐 Ready to Enter the Virtual World?
From theory to reality—discover how digital twins are reshaping the future of engineering.
👉 Explore Trail 2: Smart Digital Twins→