The history of artificial intelligence has been, in many ways, a story of scaling. Larger datasets. Bigger models. More parameters. More compute. Each leap in size brought with it a leap in capability—at least for a while. This empirical trend was codified into what became known as scaling laws: the idea that performance improves predictably as model size, training data, and compute increase. Like Moore's Law before it, scaling laws offered a seductive clarity: if you want better models, just make them bigger.
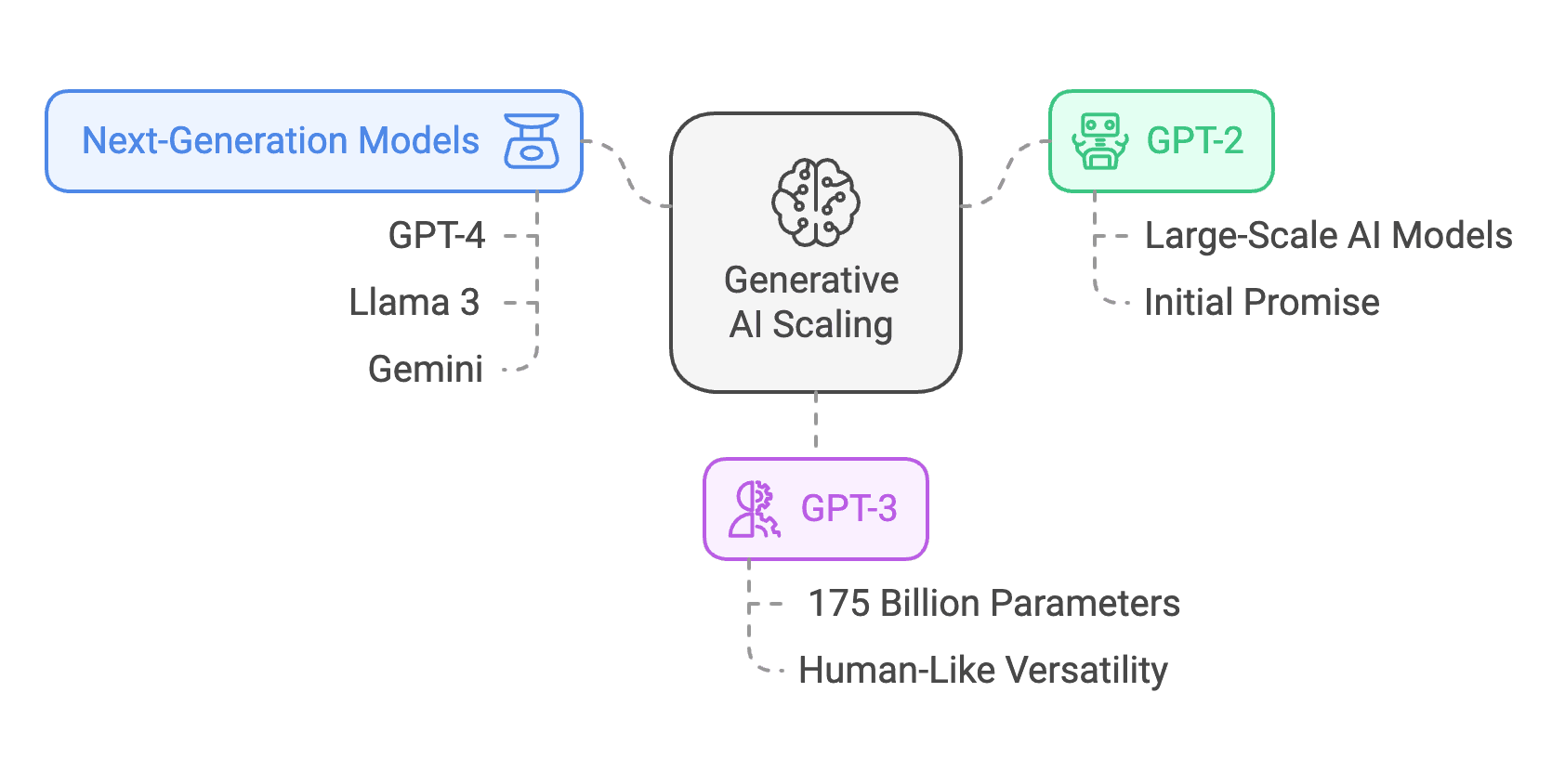
Figure 1: The Scaling Revolution: How Bigger AI Models Unlock Greater Intelligence
Generative AI scaling has driven breakthroughs from GPT-2 to next-gen models like GPT-4, Llama 3, and Gemini, proving that size, architecture, and efficiency shape AI's evolving capabilities.
(Click image to view full size)
For a time, that was true. But like all laws in the real world, scaling comes with friction. And as we push the boundaries of what's possible, we're beginning to see not a wall, but a curve: one that bends away from infinite gains and toward diminishing returns.
The Diminishing Returns of Scale
Consider GPT-4. Training this model reportedly required energy equivalent to what several hundred households consume in a year. Meta's Llama 3? A $500 million training budget. The hunger for scale isn't just computational—it's financial, environmental, and infrastructural.
Yet what do we get for this investment? Each new generation delivers less bang for its parameter buck. Performance gains taper. Training costs explode. And perhaps most concerning of all, the data well is running dry. High-quality human-generated datasets are increasingly scarce, forcing researchers to rely on synthetic data generated by the very models they're trying to improve.
This self-referential loop introduces new risks: amplification of biases, loss of originality, and degradation of reliability. Meanwhile, the so-called "black box" nature of these large models—their inscrutability—remains unsolved. In high-stakes fields like aerospace or healthcare, trust demands transparency. Bigger models don't always inspire more confidence.
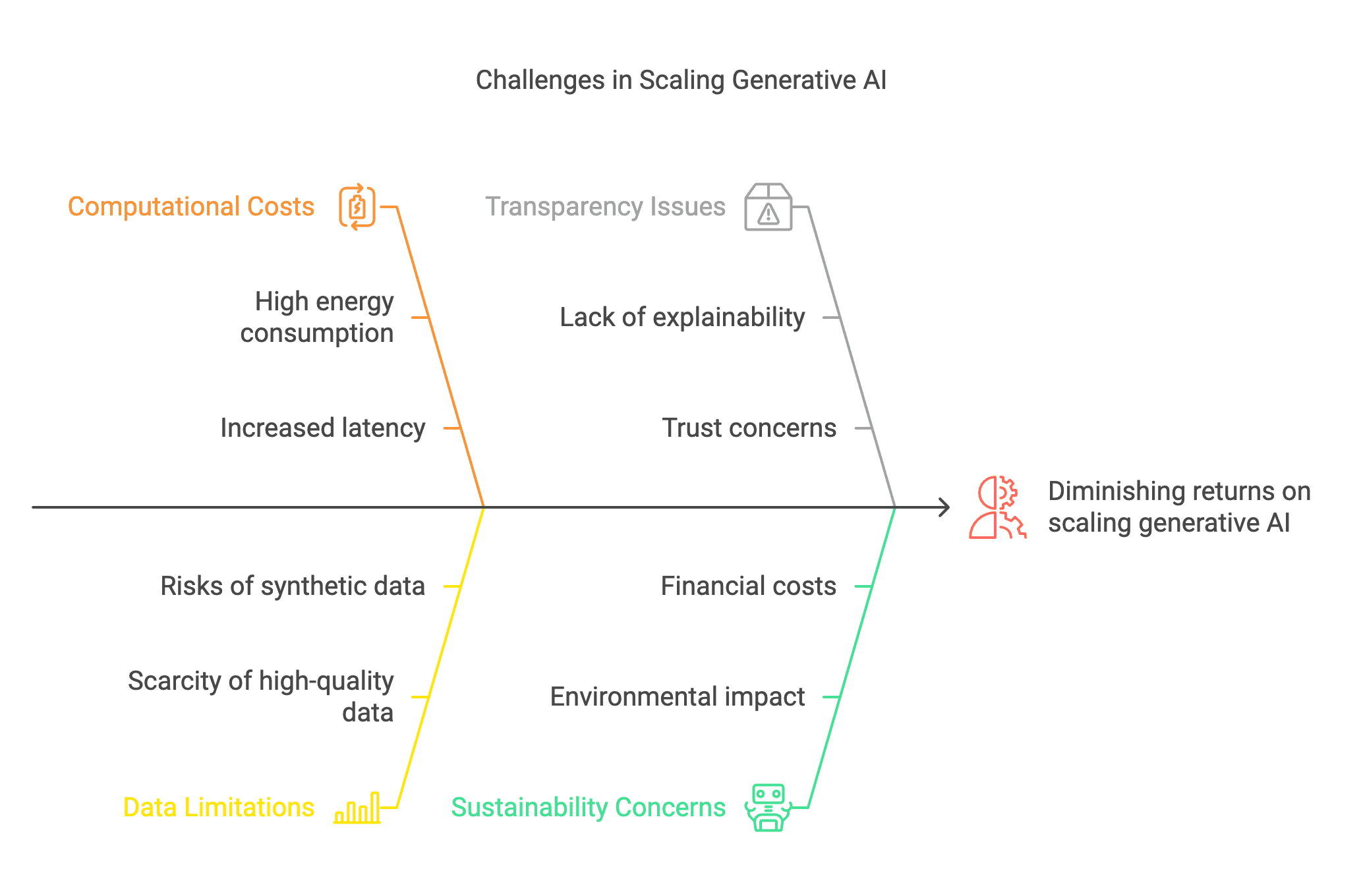
Figure 2: The Hidden Costs of Scaling: When Bigger No Longer Means Better
As generative AI scales, challenges like high energy consumption, data scarcity, and diminishing returns demand a shift from brute-force expansion to smarter, more efficient architectures.
(Click image to view full size)
Enter Efficiency: The DeepSeek Example
DeepSeek V3/R1 is a case study in smarter scaling. While it boasts 671 billion parameters, it doesn't activate them all at once. Using a Mixture-of-Experts (MoE) architecture, it selectively engages only a subset of its model per token—12.9 billion active parameters at any time. The result? A staggering 11× efficiency gain compared to traditional dense models.
Training DeepSeek cost just $5.576 million. That's not a typo.
Advanced techniques like multi-token prediction and auxiliary-loss-free load balancing further sharpen its edge. In a world obsessed with "bigger," DeepSeek makes a compelling argument for smarter.
Hardware and Software Breaking Points
"AI's hunger for power has outgrown the tools we built to feed it. As models swell to trillions of parameters, the hardware arms race becomes a battle of ingenuity."
As models balloon in size, the physical infrastructure groans under the weight. CPUs and GPUs—originally built for general-purpose tasks—struggle to keep pace. Specialized hardware like TPUs (Tensor Processing Units) and LPUs (Learning Processing Units) help, but they too face thermal limits, memory bottlenecks, and skyrocketing power consumption.
Software, meanwhile, has its own pain points. The Transformer architecture, introduced in "Attention Is All You Need," remains dominant but is beginning to show its age. Its self-attention mechanism scales quadratically with input length, making long-context processing computationally expensive.
Attempts to address this include:
Transformer-XL
Introduces segment-level recurrence and relative positional encoding, enabling the model to capture dependencies over much longer sequences without fragmenting context.
Reformers
Use locality-sensitive hashing to reduce attention complexity from O(L2) to O(L log(L)), making transformers significantly more efficient for long inputs.
Differential Transformers
Mimic noise-canceling mechanisms by filtering out irrelevant context, improving attention precision while mitigating issues like hallucination or in-context instability.
Each adaptation is a patch on a powerful, but aging engine. Transformers may still dominate today, but challengers are emerging.
New Architectures on the Horizon
The race beyond Transformers is already underway:
State-Space Models (SSMs)
The "Hungry Hungry Hippos" (H3) model exemplifies the potential of state-space models in efficient sequence processing. SSMs offer dual computational pathways—recurrence or convolution—allowing for linear or near-linear scaling with sequence length. This flexibility makes H3 particularly promising for tasks requiring long-context processing, where traditional Transformers face quadratic scaling bottlenecks.
Enhanced RNNs
Recent advancements breathe new life into Recurrent Neural Networks (RNNs), once considered overshadowed by Transformers. Augmentations like Retrieval-Augmented Generation (RAG) and lightweight attention mechanisms have narrowed the performance gap. A notable study, "RNNs are not Transformers (Yet)", demonstrated that a single Transformer layer integrated into RNNs significantly enhances in-context retrieval, enabling RNNs to excel in tasks traditionally dominated by Transformers.
Sub-Quadratic Scaling Models
Sub-quadratic architectures such as the Monarch Mixer (M2) exemplify the drive for more efficient scaling. M2 leverages Monarch matrices to optimize both sequence length and model dimension, achieving competitive results while avoiding the computational inefficiencies of Transformers.
The goal isn't just performance. It's explainability. It's efficiency. It's sustainability.
Artificial General Intelligence (AGI): Scale or System?
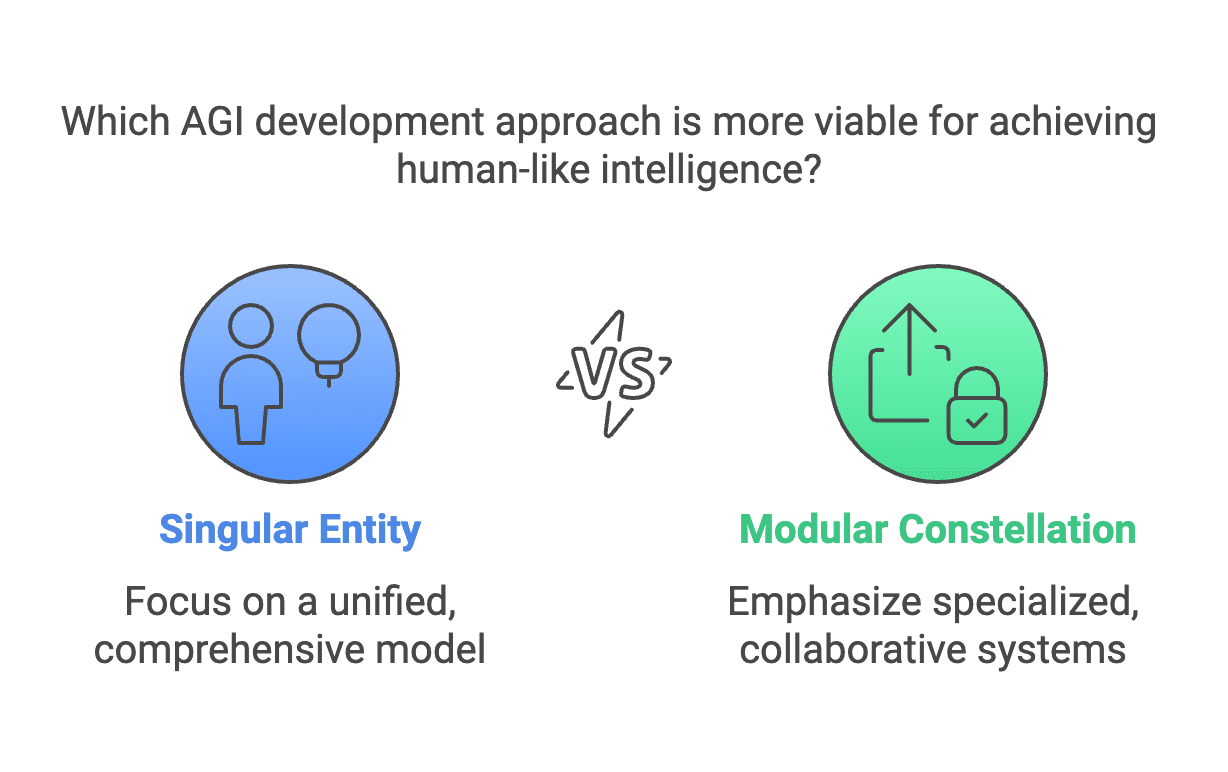
Figure 3: PAGI Development Approaches: Singular Entity vs. Modular Constellation
Should AGI be built as a singular, all-encompassing model, or as a modular constellation of specialized systems? The monolithic approach aims for a unified intelligence, while the modular vision mirrors human collaboration—combining domain-specific agents to achieve broader adaptability.
(Click image to view full size)
The quest for Artificial General Intelligence (AGI) reframes the question. Is AGI a single, massive model? Or is it an ecosystem—a modular constellation of expert agents working in harmony?
The latter is gaining favor. Think of it as an AI "orchestra" rather than a soloist. Each model contributes its domain-specific intelligence. Together, they perform symphonies no one model could compose alone. It mirrors human teams. And it's far more scalable than brute-force gigantism.
Reasoning, Creativity, and the Brain
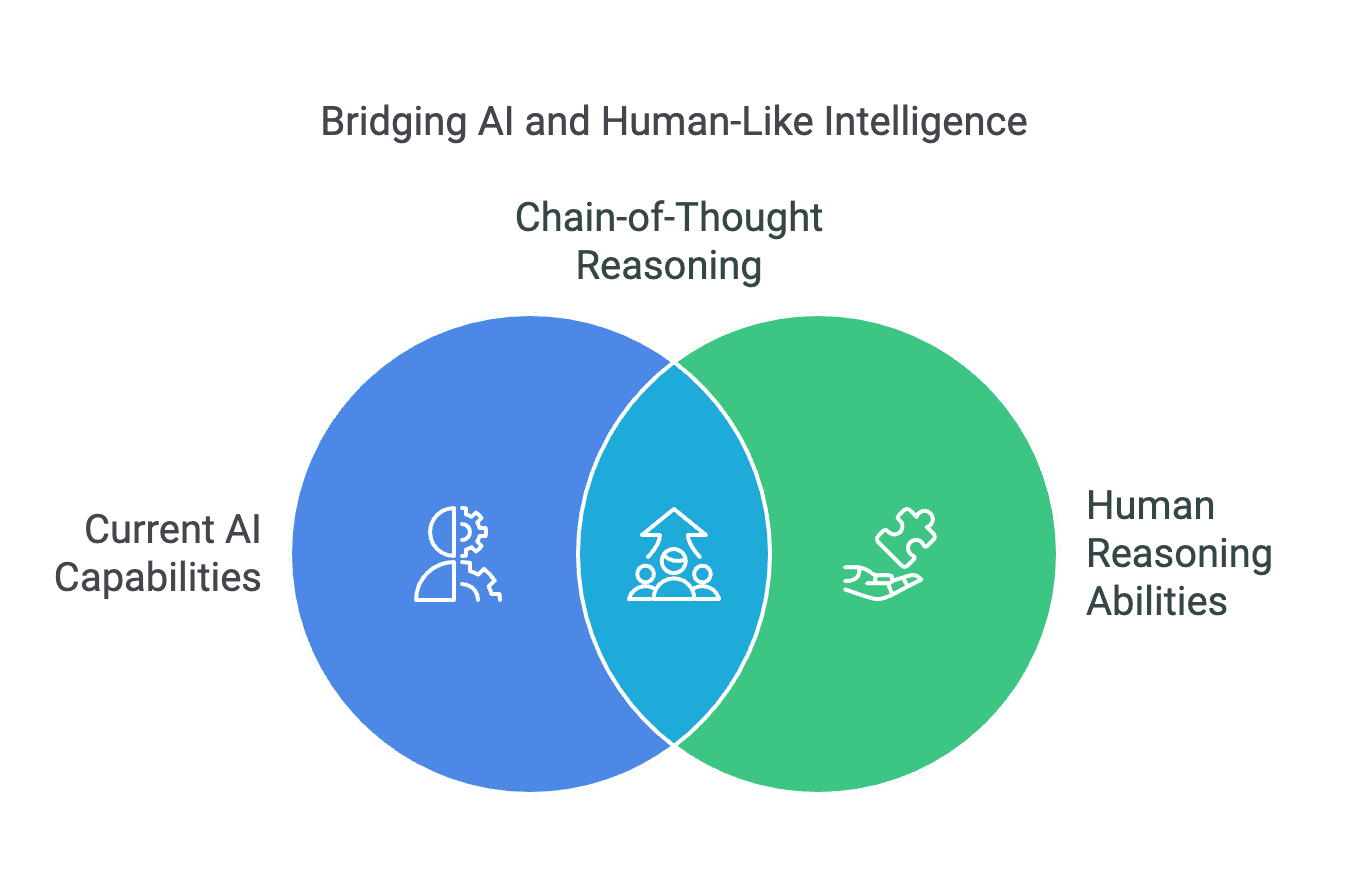
Figure 8: Bridging AI and Human-Like Intelligence with Chain-of-Thought Reasoning
AI excels at pattern recognition, while humans rely on logical reasoning. The emerging approach of Chain-of-Thought (CoT) Reasoning helps AI decompose complex problems into incremental steps, mimicking human-like thinking. Can this method bridge the gap between current AI capabilities and true AGI?
(Click image to view full size)
Real intelligence isn't just recall. It's reasoning. Abstraction. Creative synthesis. Chain-of-thought prompting has shown that breaking down problems into logical steps improves outcomes. But even this is a shallow imitation of how the brain works.
To close the gap, researchers are exploring:
- •Analogical Reasoning: Finding insight in unrelated ideas.
- •Controlled Randomness: Sparking creativity through noise.
- •Memory Integration: Blending short- and long-term memory for richer understanding.
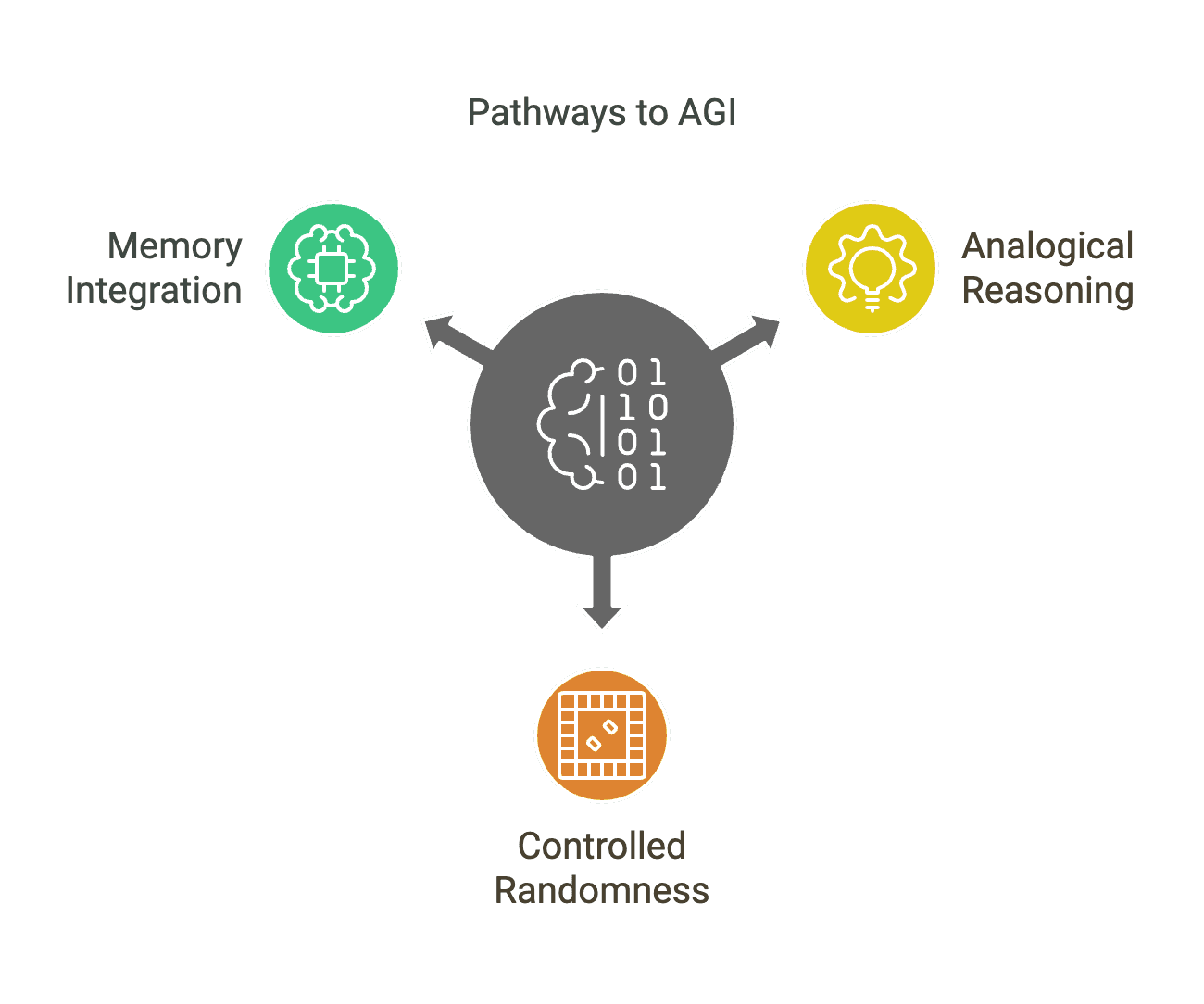
Figure 9: Pathways to AGI: Emulating the Brain's Cognitive Synergy
Achieving AGI may require mimicking the brain's cognitive flexibility—leveraging analogical reasoning for creative leaps, controlled randomness for unconventional problem-solving, and memory integration for refined decision-making.
(Click image to view full size)
Compact Intelligence: The Smarter Frontier
Yann LeCun's JEPA (Joint Embedding Predictive Architecture) is part of a movement toward compact, energy-efficient, generalizable models. Like DeepSeek, it prioritizes how intelligence is structured, not just how much of it there is.
Curriculum learning, hybrid architectures, and symbolic reasoning all point toward a future where scaling is no longer the dominant game. Smarter beats bigger. Quality over quantity.
Final Thought
The arms race of scale gave us miracles. But it also gave us monsters: bloated models, opaque decisions, and environmental tolls. The next era of AI will not be won by those who build the biggest machines. It will be led by those who build the most elegant ones.
Because in the end, intelligence isn't just about size. It's about grace.