Introduction: The End of Scale as We Know It
For the past decade, the AI frontier has been synonymous with scale. Larger models, more data, more compute. But just as Moore's Law begins to falter in silicon, a parallel slowdown is emerging in AI: the law of diminishing returns. As we push beyond hundreds of billions of parameters, the gains are tapering, and the costs—both economic and environmental—are mounting.
This essay explores a smarter trajectory for AI, where efficiency, modularity, and trust eclipse brute force. It dives into emerging architectures, smarter training strategies, and the shifting role of engineers from model builders to system orchestrators.
Scaling Laws: The Highs and the Hurdles
Scaling laws in AI—which predict performance improvement as model size increases—held remarkably well throughout the 2010s. More data, larger models, and more compute led to better accuracy, broader capabilities, and a surge in generative power. Let's dive deeper into the principle of scale.
In 2019, OpenAI's GPT-2 showcased the emerging promise of large-scale AI models. But it was GPT-3, with its 175 billion parameters, that epitomized the transformative potential of scale. The investment was staggering—billions poured into autoregressive models—yet the results were undeniable: fluid language generation, nuanced context understanding, and applications spanning industries. GPT-3 proved that sheer size could unlock human-like versatility, providing solutions across coding, design, and conversational tasks.
The story didn't stop there. The principle of scale has been further validated by the emergence of next-generation models, each pushing the boundaries of capability:
GPT-4
OpenAI's GPT-4, believed to contain over 1 trillion parameters—an exponential leap from GPT-3's 175 billion—demonstrates what scaling can achieve.
- It achieves 40% higher accuracy on OpenAI's internal factual performance benchmark compared to GPT-3.5.
- In standardized evaluations, GPT-4 moved from bottom 10% performance (GPT-3.5) to the top 10%, underscoring its gains in reasoning and problem-solving.
- These advancements show that scale alone has driven substantial improvements, particularly in factual consistency, reasoning, and general task proficiency.
Llama 3
Meta's Llama 3 exemplifies how scaling and refined architectural strategies can drive superior performance. Llama 3 employs a dense Transformer architecture, optimized for scale and efficiency. Its largest model, with 405 billion parameters and a 128K token context window, represents a significant leap in capacity while maintaining architectural simplicity.
Trained on a 15 trillion token multilingual corpus, Llama 3 demonstrates enhanced capabilities in multilinguality, coding, reasoning, and tool usage. These advancements are evident in benchmark performance: the 8B version outperforms models like Gemma 9B and Mistral 7B on tasks such as MMLU, HumanEval, GSM8K, and MATH, underscoring its efficiency in handling diverse, complex challenges.
Architectural innovations include:
- 126 Transformer layers, a 16,384-dimensional token representation, and 128 attention heads in the 405B model.
- Modular integration for processing image, video, and speech inputs, signaling progress toward a unified multimodal framework.
While the multimodal capabilities are still under development, Llama 3's core strength lies in its dense architecture and optimization. Llama Guard 3, a safety layer for input-output moderation, further enhances its reliability, making it suitable for high-stakes applications.
Llama 3's performance parallels leading models like GPT-4 across multiple domains. Its success illustrates how intelligent scaling—not brute-force expansion—can yield state-of-the-art results with nuanced efficiency.
Gemini
Google's Gemini models extend the principle of scale into multi-modal capabilities. By integrating text and visual data seamlessly, Gemini showcases the advantages of scaling models beyond language tasks.
Gemini excels in combining and interpreting textual, visual, and symbolic inputs, setting new benchmarks in multi-modal AI performance.
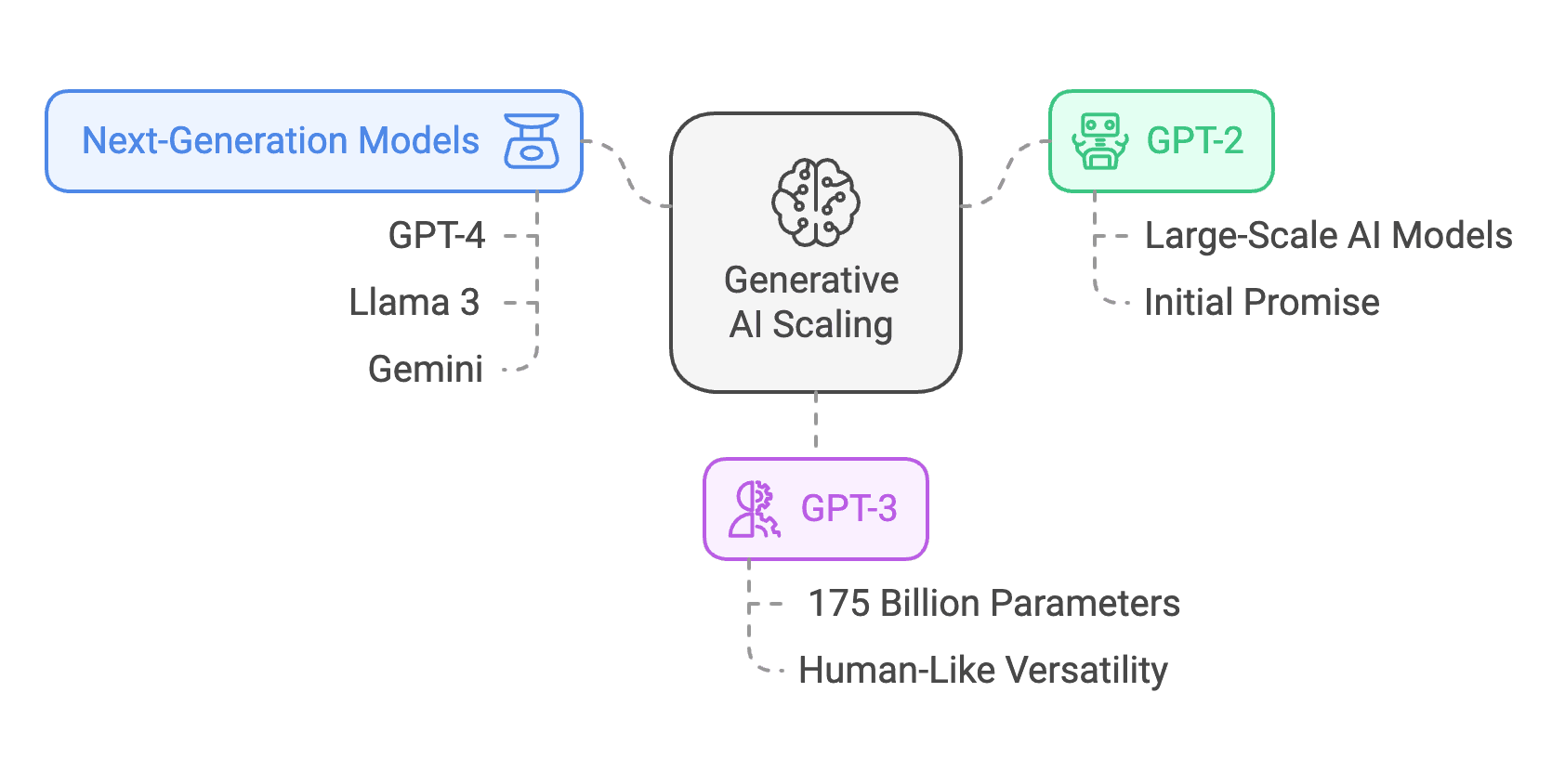
Figure 1: The Scaling Revolution: How Bigger AI Models Unlock Greater Intelligence
Generative AI scaling has driven breakthroughs from GPT-2 to next-gen models like GPT-4, Llama 3, and Gemini, proving that size, architecture, and efficiency shape AI's evolving capabilities.
(Click image to view full size)
But now the curve is bending.
Training GPT-4, for instance, required compute resources so vast that a single run consumed the energy of hundreds of households per year. Meta reportedly spent over $500 million on LLaMA 3. These costs are not just financial; they are ecological and ethical.
Worse, we are running out of clean, diverse data. As the internet's high-quality training material gets exhausted, researchers are turning to synthetic data—AI training on AI—a loop that risks bias amplification, hallucinations, and recursive errors.
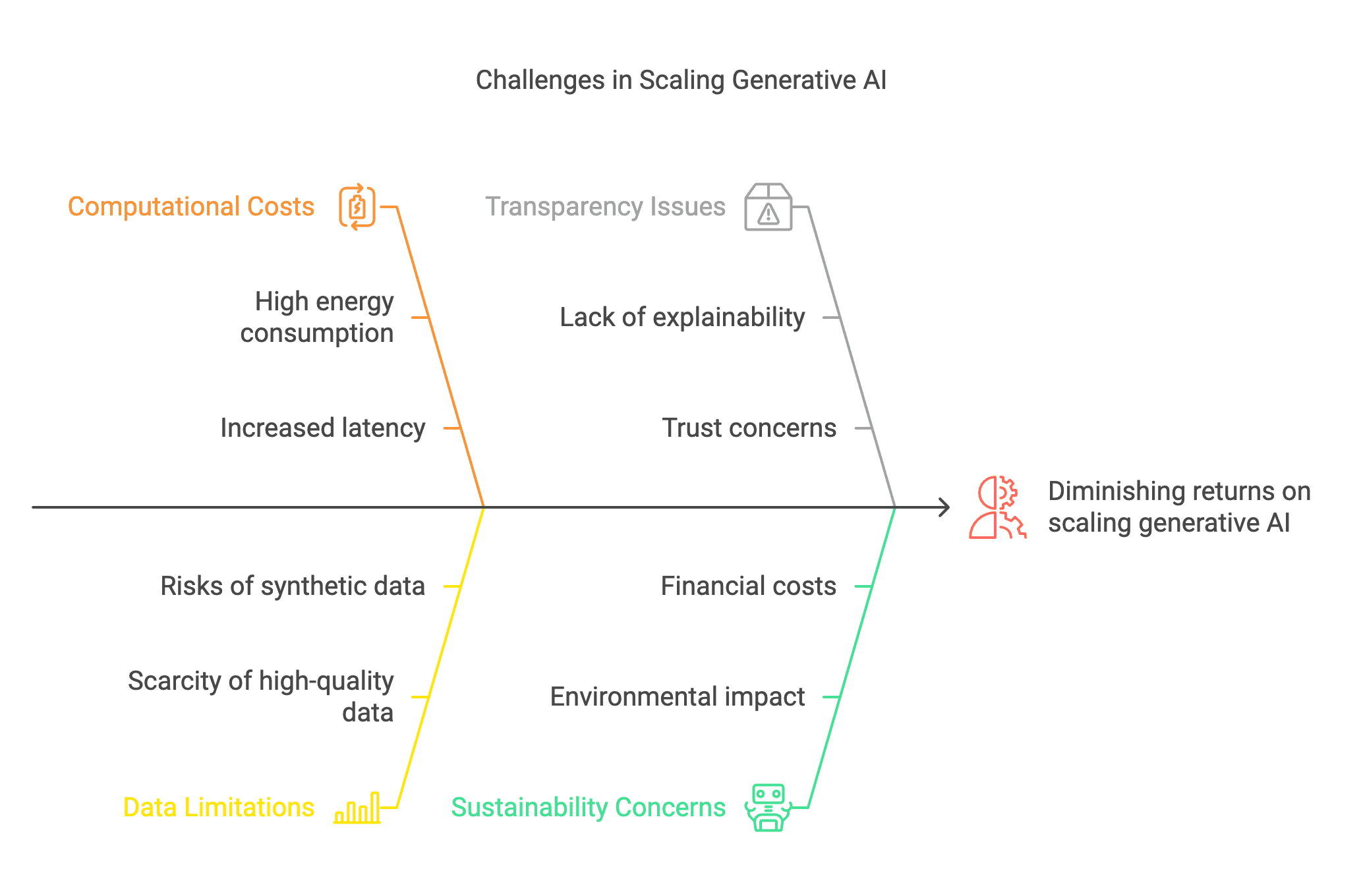
Figure 2: The Hidden Costs of Scaling: When Bigger No Longer Means Better
As generative AI scales, challenges like high energy consumption, data scarcity, and diminishing returns demand a shift from brute-force expansion to smarter, more efficient architectures.
(Click image to view full size)
The era of "bigger is better" is ending. A new path must begin.
Architectures That Think Small to Go Big
DeepSeek V3 exemplifies this shift. Rather than activating all 671 billion parameters at once, it uses a Mixture-of-Experts (MoE) approach, selectively activating only a portion per token. The result: 11× computational efficiency at a fraction of the cost. Training DeepSeek cost just $5.576 million. For comparison, GPT-4's compute bill is estimated in the hundreds of millions. Techniques like auxiliary-loss-free balancing and multi-token prediction further reduce waste without sacrificing accuracy. This is not just optimization; it's architectural reinvention.
Yann LeCun's Joint Embedding Predictive Architecture (JEPA) furthers the cause. JEPA seeks to learn compact, generalizable representations rather than massive pattern overlays. This unlocks high performance with less data and compute—and opens the door to more sustainable, accessible AI.
Together, these models illustrate a new mantra: compact is capable.
Training Smarter: The Shift from Brawn to Brains
"AI's hunger for power has outgrown the tools we built to feed it. As models swell to trillions of parameters, the hardware arms race becomes a battle of ingenuity. Compact representations are redefining how AI systems process information."
Curriculum learning borrows from human education. Start with simple problems, and gradually move toward complex ones. This improves data efficiency and reduces overfitting.
Hybrid approaches combine deep learning with symbolic logic or reinforcement learning. This enables better reasoning and decision-making, especially in fields like robotics or autonomous systems.
Efficiency is no longer a bonus—it is a necessity. Compact models, smarter training, and hybrid logic are the only sustainable ways forward.
Democratization: Open-Source as a Scalable Ethos
Models like LLaMA, Mistral, and DeepSeek have embraced open-source, lowering barriers for global researchers and developers. This stands in contrast to closed giants like GPT-4 and Gemini.
The move toward openness also reflects the growing recognition that AI cannot be monopolized. Responsible development requires transparency, community scrutiny, and shared innovation.
Engineers: From Model Builders to System Orchestrators
This shift toward intelligence over scale redefines the role of engineers. Generative AI is no longer just a tool—it's a collaborator.
- In routine tasks, AI acts as an assistant.
- In creative iteration, it becomes an augmenter.
- In narrow, defined domains, it may even act autonomously—as an agent.
But the engineer remains the architect of trust. They validate, interpret, and refine AI outputs. In high-stakes domains—infrastructure, aerospace, medicine—AI must assist, not decide.
Trust, not scale, is the new frontier.
Evaluating Generative AI: A Practical Framework
"Generative AI's power lies not just in what it can do, but in how well it can deliver. Precision, cost, speed, and impact—these are the true measures of its value in engineering's evolving landscape."
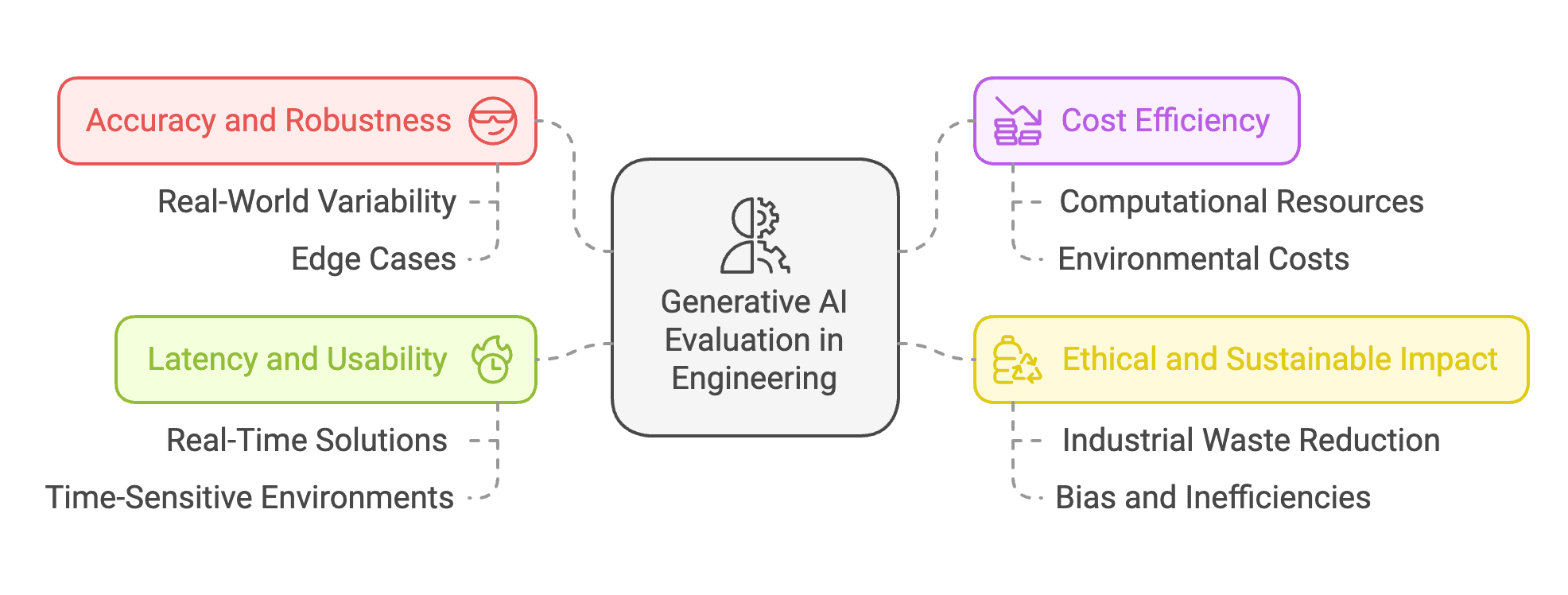
Figure 1: Evaluating Generative AI in Engineering
Generative AI in engineering is evaluated based on accuracy and robustness, cost efficiency, ethical and sustainable impact, and latency and usability to ensure reliability, practicality, and alignment with real-world constraints.
(Click image to view full size)
To guide adoption, engineers can assess generative AI along four axes:
Accuracy and Robustness
Precision is non-negotiable in engineering. Can generative AI produce solutions that account for real-world variability, edge cases, and unforeseen conditions? For example, in structural engineering, does the AI account for unexpected stress loads while preserving safety margins, or does it falter under conditions it hasn't explicitly seen?
Cost Efficiency
High performance often comes at a steep price. Training large-scale models requires massive computational resources—one training run for models like GPT-4 can consume as much energy as hundreds of homes annually. Does the value delivered by AI justify the economic and environmental costs? Can smaller, more efficient models achieve similar outcomes without sacrificing quality?
Ethical and Sustainable Impact
Generative AI must align with broader societal goals. Does it reduce industrial waste, minimize carbon footprints, and promote equitable access? Or does it perpetuate biases, amplify inefficiencies, or exacerbate environmental challenges? As datasets saturate, reliance on AI-generated synthetic data raises further questions: can these models avoid recursive errors or hidden biases that undermine reliability?
Latency and Usability
Engineering workflows often demand real-time or near-real-time solutions. From autonomous vehicles to energy grid management, a split-second delay can mean catastrophic failure. Generative AI must balance speed and accuracy, especially in time-sensitive environments.
And along a spectrum of collaboration:
Assistant (low-stakes automation)
At its most basic, generative AI performs well-defined, low-stakes tasks—generating design drafts, running simulations, or automating repetitive calculations. Engineers oversee the work closely, ensuring outputs meet project requirements and quality standards. AI, in this role, is a reliable tool that saves time and reduces cognitive load.
Augmenter (human-in-the-loop creativity)
As tasks grow more complex, AI becomes a creative collaborator. It contributes insights for resource allocation, system optimization, or multivariable trade-offs. Engineers rely on AI to explore possibilities, but they retain ultimate decision-making authority. The synergy of human creativity and AI precision leads to solutions that neither could achieve alone.
Peer/Agent (specialized autonomy, with clear guardrails)
In specialized, narrowly defined domains, generative AI operates with minimal human input, taking on tasks that require consistency and speed. For example, AI-driven diagnostic systems in manufacturing or autonomous drone navigation may function with little oversight. Yet this level of autonomy raises critical questions:
Who is accountable when AI fails? What boundaries must remain in place to preserve safety, fairness, and human judgment?
Conclusion: Intelligence, Not Size, Defines the Future
The smartest AI of the future will not be the largest. It will be the most thoughtful, efficient, and aligned. It will learn like a student, reason like a colleague, and collaborate like a teammate. In that world, the role of the engineer becomes more vital, not less. They are not outpaced by machines—they are empowered by them.
The road to AGI is not paved with scale. It is carved by design.
AI Terminology Glossary
New to AI concepts? Expand the terms below for quick definitions to help you navigate this content.