Historical Perspective
From Turing's Question to the Generative Leap — How AI Rewrote Engineering's DNA
Engineering has long served as humanity's instrument of ambition—translating abstract ideas into material reality. It built aqueducts to defy terrain, cathedrals to defy gravity, and rockets to defy Earth itself. But until recently, engineering was guided by a single covenant: human mastery over deterministic rules. That covenant has been rewritten.
The turning point traces back to 1950, when Alan Turing posed his deceptively simple question: "Can machines think?" It cracked open a new domain—Artificial Intelligence—and five years later, the 1956 Dartmouth Conference gave it a name and a mandate. For a moment, the future felt imminent. Machines would learn, reason, even understand. But early AI stumbled under its own weight. Limited data, expensive compute, and brittle logic trees led to the "AI Winters" of the 1970s and 1980s, when hype froze into skepticism, and engineering doubled down on what it knew best: equations, heuristics, and controlled systems.
Then the millennium tipped, and the equation shifted. The rise of the internet poured data into every corner of the world. Graphics Processing Units (GPUs)—originally designed for video games—were repurposed to train deep learning models. The machine no longer needed to be told what to do. It could learn. This ushered in the golden trifecta of Machine Learning (ML): supervised, unsupervised, and reinforcement learning.
Supervised Learning gave machines a memory of the past—training them on labeled data to classify tumors, detect defects, or predict failure points in industrial systems. It didn't invent, but it scaled human expertise with breathtaking fidelity.
Unsupervised Learning, in contrast, gave machines intuition. Without explicit labels, they could cluster patterns, unveil hidden structures, and discover relationships invisible to the human eye—whether in sensor data from a jet engine or usage patterns across a smart grid.
Then came reinforcement learning, an algorithmic echo of how humans learn by doing. It unlocked strategic reasoning. In 2016, DeepMind's AlphaGo stunned the world—not by winning against the world champion of Go, but by playing differently, inventing moves never seen before in 2,500 years of recorded games. This wasn't brute force. It was a glimpse of machine creativity.
But if AlphaGo hinted at the future, Generative Adversarial Networks (GANs) pulled it forward. Introduced in 2014, GANs turned neural networks against each other—one generating, one judging—in a high-stakes duel of imagination. The result? Photorealistic faces, synthetic medical images, even entirely new fashion collections. It was engineering that could dream.
And then, in 2017, came the architecture that changed everything: the Transformer, introduced by Vaswani et al. in "Attention Is All You Need". Transformers redefined how machines handle language, vision, and code by replacing sequential logic with parallel self-attention. They captured context not just locally, but across entire sequences—turning fragmented signals into coherent understanding.
From this, OpenAI's GPT series was born. GPT-3, with 175 billion parameters, wasn't just a large model—it was a linguistic symphony. It could write, reason, and riff like a human. And in late 2022, ChatGPT—built atop GPT-3.5—reached 100 million users in just two months, the fastest tech adoption in history. The interface was deceptively simple. The implications were seismic.
This wasn't just a computational shift—it was a philosophical one. For centuries, engineering meant solving deterministic problems with fixed tools. Generative AI disrupted that lineage. Now, engineers could co-create with a system that learns, adapts, and surprises. As Google's Sundar Pichai observed in 2023, "Generative AI will revolutionize every aspect of engineering, from design to production." Not a metaphor. A manifesto.
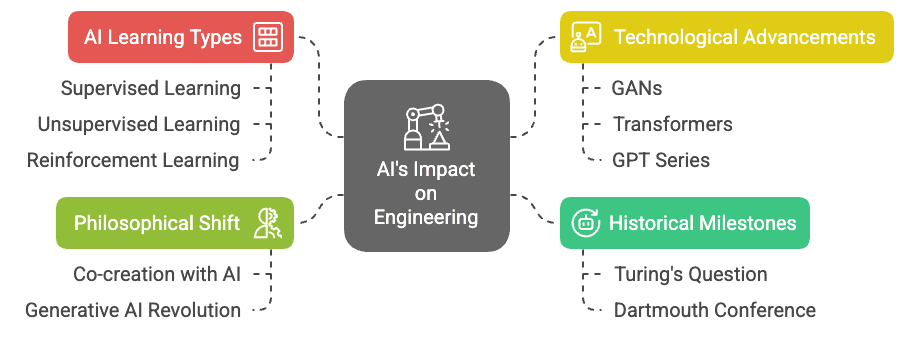
Figure 1: Mapping the Pillars of AI's Evolution in Engineering
AI's impact on engineering spans learning types, technology, philosophy, and historical milestones.
(Click image to view full size)
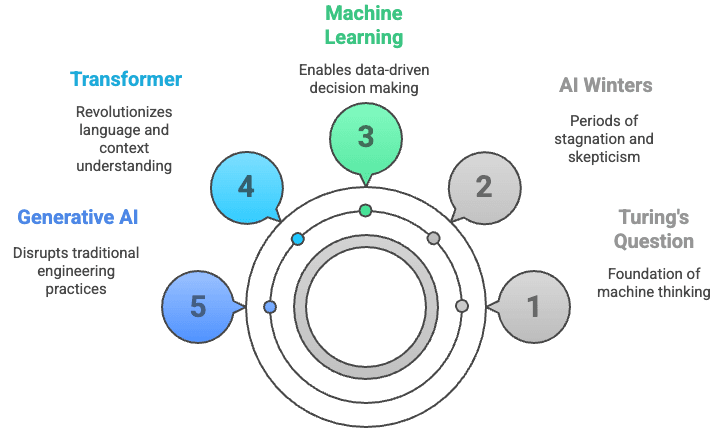
Figure 2: Timeline of Milestones in AI and Engineering
Five major stages in the evolution of AI in engineering: from Turing's foundational question and the setbacks of AI Winters, to the rise of machine learning, Transformers, and the disruptive potential of Generative AI.
(Click image to view full size)
Yet the revolution is not frictionless. Model sizes ballooned—from millions to billions to trillions of parameters—bringing environmental costs measured in megawatt-hours and megatons of CO₂. Trust became an issue, too. How do you validate outputs from a model you can't fully interpret? How do you test an AI-designed bridge that no human would have dared to imagine?
The French engineering tradition—grounded in Cartesian rigor and scientific skepticism—may offer a timely counterbalance. It could remind the world that generative doesn't mean unchecked. AI must not only generatenovelty, but validity.
And so, engineers now find themselves at an inflection point. No longer just calculators or builders, they must become co-pilots, curators, and custodians—balancing machine creativity with human responsibility.
This text is your map through that new terrain. What follows is not just a curriculum. It is an invitation: to rethink your tools, your methods, and even your mindset. Before we embark on the first trail—Optimization—let's explore what generative AI really is, technically and philosophically.
Technical Overview of Generative AI
Beyond Automation: How Generative AI Rewired the Logic of Engineering
"Generative AI doesn't just refine engineering—it reinvents it. By turning machines into collaborators, it transforms the impossible into the inevitable."
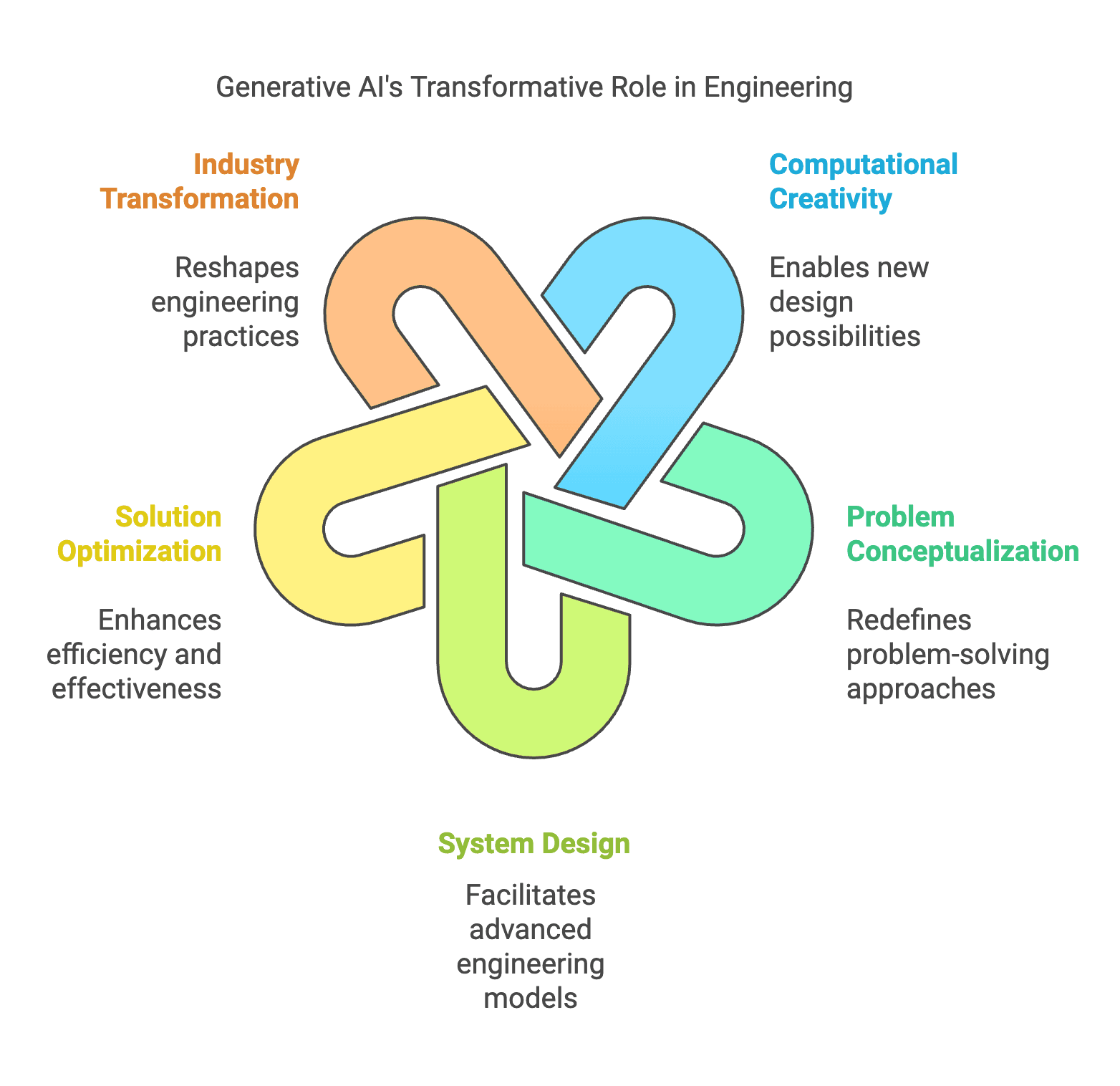
Figure 3: Generative AI: A Paradigm Shift in Engineering
Generative AI transforms engineering by redefining problem-solving, enhancing computational creativity, optimizing solutions, and reshaping industries through advanced system design.
(Click image to view full size)
At first glance, generative AI might seem like just another clever tool—an automation assistant that predicts text, generates images, or writes code. But that surface simplicity hides a radical shift. Generative AI doesn't just speed up engineering. It reconfigures its logic.
Traditional engineering relies on determinism. You begin with known variables, apply well-understood equations, and trace a linear path to a solution. This approach gave us airplanes, power grids, and microchips—but it also anchored us to the limits of human foresight. Engineering under this model was defined by rules, and progress meant refining them.
Generative AI introduces a new paradigm: systems that don't follow fixed rules, but learn them—adaptively, contextually, and creatively. Rather than designing the solution, the engineer now designs the conditions under which a model generates solutions—some expected, some unthinkably original.
This shift emerges from a powerful blend of three core machine learning paradigms. Alone, each is potent. Together, they form the cognitive backbone of generative AI.
1. Supervised Learning – Scaling Human Expertise
Imagine an apprentice studying under a master engineer. The master provides annotated blueprints, failed prototypes, and optimal solutions. The apprentice mimics, internalizes, and eventually generalizes these lessons to new designs.
That's supervised learning: machines trained on labeled data to reproduce patterns humans already understand. In engineering, this paradigm has been transformative. Supervised models now detect microfractures in materials more reliably than expert inspectors. They classify structural safety risks, predict energy demand, and identify system faults in real time. In aerospace, they streamline quality assurance; in medicine, they classify tumors with near-clinical accuracy.
But supervised learning, for all its power, is inherently conservative. It can reproduce brilliance, but it cannot surpass it. It's bounded by the scope of its training data—and the limits of what humans already know.
2. Unsupervised Learning – Discovering the Unknown
If supervised learning is mentorship, unsupervised learning is exploration without a map. Here, the machine looks for structure in unlabeled data—clustering, compressing, correlating—searching for hidden signals humans might never spot.
In engineering, this opens new frontiers. Imagine sensors embedded in a suspension bridge generating terabytes of vibration data. An unsupervised model can sift through this noise, detect subtle anomalies, and identify stress points long before visible cracks appear. It can analyze factory output, usage patterns, or network behaviors—revealing optimizations no human formulated in advance.
One of the most striking innovations from this paradigm is the Generative Adversarial Network (GAN). Invented by Ian Goodfellow in 2014, GANs pit two neural networks against each other: a generator that creates, and a discriminator that critiques. This adversarial loop produces outputs of uncanny realism—photorealistic faces, synthetic satellite images, even plausible aerodynamic structures.
GANs showed something profound: AI could generate new content, not just reproduce old patterns. It could suggest solutions never before seen—not by searching a database, but by inventing. Yet unsupervised learning, like creativity without constraint, sometimes goes off-course. It produces results that are novel, but not always useful. That's where reinforcement learning enters the scene.
3. Reinforcement Learning – Learning by Doing
In reinforcement learning, the machine becomes an agent in a simulated environment. It takes actions, observes results, and receives rewards or penalties. It learns like a chess player: not by being told the rules, but by playing—losing, winning, adjusting.
Engineering has always embraced this trial-and-error logic. Think of design loops, prototyping, or stress testing. Reinforcement learning compresses these cycles from months into minutes. It can train a robotic arm to optimize its grip, a drone to navigate turbulent winds, or a logistics network to self-adjust delivery routes in real time.
DeepMind's AlphaGo didn't just win by memorizing human games—it discovered new strategies, expanding the game itself. When this learning is transferred to engineering, it's not just about efficiency. It's about reimagining what's possible.
Transformers: Scaling Intelligence Without Bottlenecks
These three learning paradigms already revolutionized AI. But they faced a common constraint: memory and context. Early models struggled to hold long sequences—whether lines of code or pages of text—forcing engineers to simplify problems into smaller chunks.
That changed with the introduction of the Transformer in 2017.
The Transformer architecture, introduced in "Attention Is All You Need", replaced sequential processing with self-attention. Rather than reading one word or token at a time, it looks at everything at once—dynamically weighting relevance across the input.
This architectural leap unlocked scalability. Suddenly, models could train on entire design documents, system logs, or engineering manuals. They didn't just parse context—they understood it. That's how GPT-3, with its 175 billion parameters, could write code, draft legal documents, and simulate human reasoning—all with surprising fluency.
The Transformer wasn't just an algorithm. It was an organizing principle for modern AI.
Mixture of Experts and the Return of Modularity
As models grew, so did their cost—financially, environmentally, and computationally. GPT-3 costs millions to train, and consumes energy rivaling that of small towns.
Enter the Mixture of Experts (MoE). Inspired by how organizations deploy teams of specialists, MoEs activate only the sub-models needed for a task. The rest remain idle. This dramatically improves efficiency without sacrificing performance—like calling on an electrical engineer instead of an entire engineering firm.
Meta, Google, and Microsoft now experiment with MoE architectures to combine scale with sustainability. They signal a return to modularity—not monolithic models, but agile, expert systems that collaborate.
Beyond Transformers: The Next Wave
Even as Transformers dominate, new architectures are emerging to address their limits. Transformers scale quadratically with input length—a major constraint in fields like fluid dynamics or climate modeling.
Alternatives include:
- State Space Models (SSMs), which model continuous systems efficiently, ideal for long-range signal processing or time-series data.
- Monarch Mixers (M2), which reduce computational load by using sparse, structured matrices—promising in fields like embedded systems or edge computing.
- Enhanced RNNs, which hybridize old sequential models with attention, improving temporal reasoning with lower memory footprints.
And on the horizon looms Yann LeCun's JEPA framework—an architecture that prioritizes world modeling over next-token prediction. Instead of answering "What comes next?", JEPA asks, "What should be true in this situation?" That's not autocomplete. That's common sense.
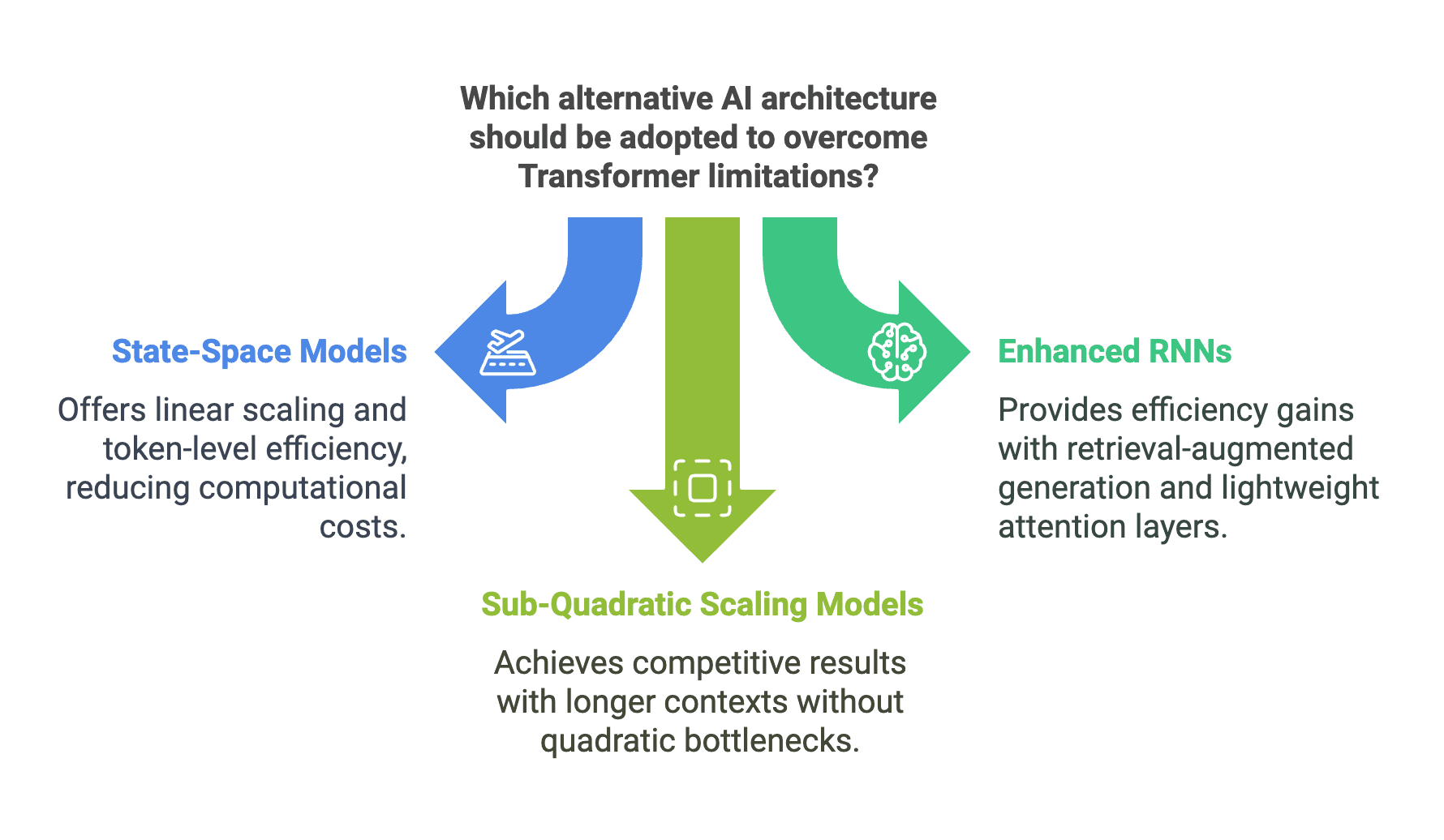
Figure 4: Beyond Transformers: Emerging Architectures for AI Scaling
New AI architectures—State-Space Models, Enhanced RNNs, and Sub-Quadratic Scaling Models—are challenging the dominance of Transformers by addressing efficiency, scaling, and explainability bottlenecks.
(Click image to view full size)
From Models to Agents – The Rise of Intentional Systems
The next frontier isn't just smarter models. It's Agentic AI—systems that act with goals, learn in real time, and operate semi-autonomously in complex environments.
Agentic AI blends learning with decision-making. It doesn't just respond—it reasons. Gartner forecasts that by 2030, over 80% of enterprise interactions could be handled by AI agents. In engineering, these agents might monitor factory floors, reconfigure energy grids, or optimize building ventilation—without human prompting.
They combine:
- Large Conceptual Models (LCMs) – for world knowledge and high-level reasoning.
- Large Quantitative Models (LQMs) – for numerical precision and simulation.
- Quantized LLMs – for lightweight, real-time inference at the edge.
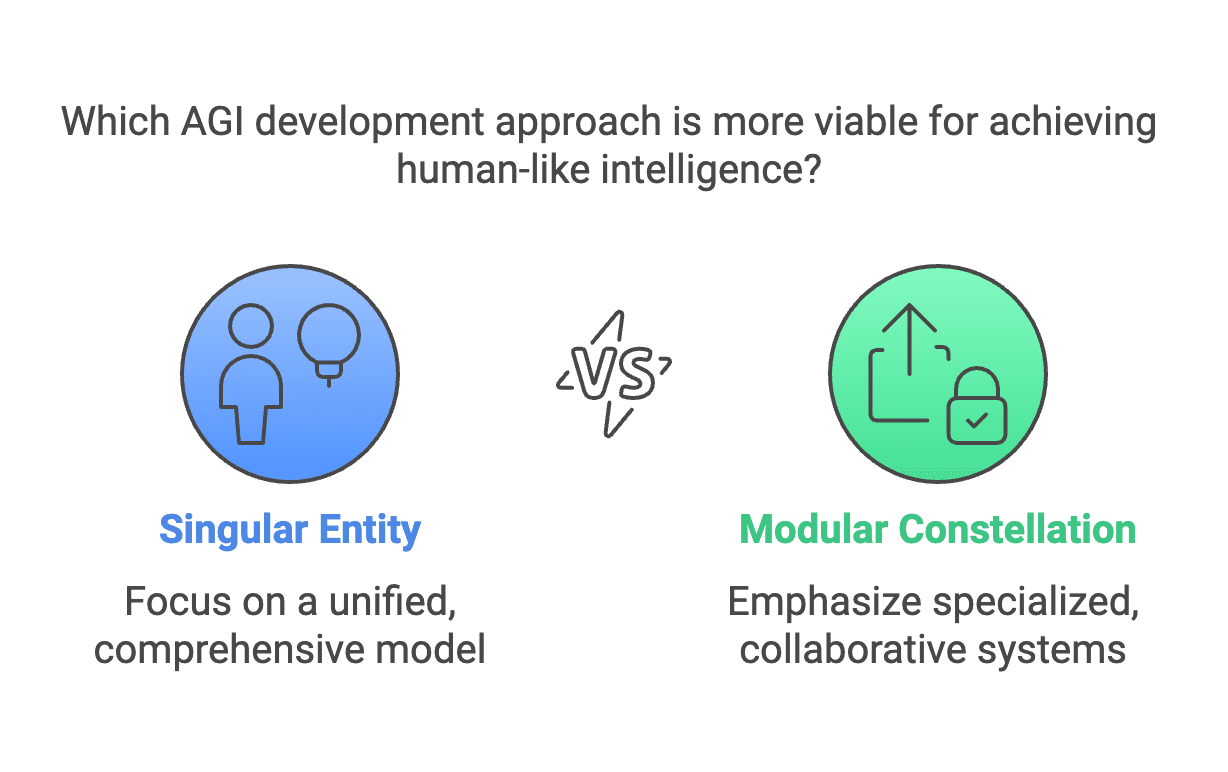
Figure 7: PAGI Development Approaches: Singular Entity vs. Modular Constellation
Should AGI be built as a singular, all-encompassing model, or as a modular constellation of specialized systems? The monolithic approach aims for a unified intelligence, while the modular vision mirrors human collaboration—combining domain-specific agents to achieve broader adaptability.
(Click image to view full size)
This evolution hints at Artificial General Intelligence (AGI)—not just narrow mastery, but adaptive, open-ended intelligence.
Yet as capabilities grow, so does the burden of responsibility. If an AI proposes a novel design for a bridge, who verifies it? Who is liable if it fails?
Conclusion – A New Logic for a New Era
Generative AI transforms engineering from a rule-based discipline into a generative, exploratory one. It redefines the engineer's role: not as the sole problem-solver, but as a co-designer of intelligence itself. It demands new tools, new workflows, and most of all, new mindsets.
What comes next is not a tutorial—but a navigation system. The trails ahead—Optimization, Smart Digital Twins, Intelligent Agents, and Sustainability—offer more than insight. They offer orientation. Because in this new terrain, maps matter. But learning how to chart your own becomes the real craft of tomorrow's engineer.
🛠️ Ready for a Smarter Future?
Discover how smarter, smaller, more intentional models are reshaping engineering's trajectory.
From efficiency to ethics, explore the path to a more sustainable AI future.
Real-World Engineering Applications
From Design to Deployment: How Generative AI Redraws the Blueprint of Engineering
For centuries, engineering was an act of disciplined imagination. You dreamt, but within constraints. You optimized, but with trade-offs. The limits were fixed: time, cost, materials, and—most of all—human cognition. No matter how brilliant the design, it lived in the engineer's head, then slowly took shape through blueprints, calculations, and prototypes.
Generative AI changes this rhythm. It accelerates engineering not by cutting corners, but by expanding dimensions—turning the constraints themselves into inputs for creativity. Suddenly, systems can generate thousands of design permutations before lunch, simulate entire ecosystems overnight, or suggest architectural solutions that echo nature more than human intuition.
But to understand this revolution, we must distinguish two concepts often conflated: Generative Design and Generative Engineering.
Generative Design – Creativity, Supercharged
Generative design emerged in the early 2010s like a new lens on an old problem. Engineers, once constrained to iterate by hand, now had software that could suggest possibilities. Feed the system a few constraints—maximum weight, load-bearing thresholds, material limits—and it produced dozens, even hundreds, of viable designs.
It was creativity at scale.
Tools like Autodesk Fusion 360 and Dassault Systèmes CATIA became co-pilots for mechanical and aerospace engineers. When Airbus sought to redesign a bulkhead component in its A320, generative design cut the part's weight by 45%, shaving kilograms off the aircraft and millions off fuel costs. General Motors, in collaboration with Autodesk, reimagined a seat bracket—traditionally made of eight components—into a single, 3D-printed lattice structure. It was 40% lighter and 20% stronger.
This wasn't about drawing faster—it was about drawing differently. The algorithm wasn't replicating known solutions. It was exploring forms never seen before, echoing coral reefs, bone structures, or fractal geometry. In architecture, firms embraced generative design to optimize natural light, airflow, and materials. The Beijing National Stadium and Zaha Hadid's sinuous buildings hinted at this aesthetic long before it became computational. Now, the software caught up with the vision.
But generative design had its ceiling. It stopped at the drawing board. The outputs were often still static, demanding human intervention to navigate complexity, feasibility, or changing requirements.
Enter Generative Engineering.
Generative Engineering – Intelligence in Motion
Where generative design stops at proposing shapes, generative engineering orchestrates systems. It weaves intelligence into the entire lifecycle—design, simulation, production, deployment, monitoring, and iteration.
It's not about one optimized part. It's about how the part interacts with supply chains, usage patterns, environmental conditions, and long-term sustainability. It's not a single recommendation; it's a continuous loop.
In 2023, Google's Vertex AI positioned itself not merely as a model deployment platform, but as an engineering partner. Its mission? Embed generative AI into every step of production pipelines. At Siemens, digital twins of factories now communicate directly with generative models. The system predicts faults before they occur, reschedules workloads autonomously, and proposes equipment configurations that would take a human team weeks to calculate.
This shift reframes the role of the engineer—from an operator of systems to a conductor of intelligence.
From Static Designs to Living Systems
Let's examine the world this creates, one industry at a time.
Urban Infrastructure – Simulating Cities Before They're Built
Take Singapore, often described as a smart city decades ahead of its peers. In 2018, it launched Virtual Singapore, a 3D digital twin of the entire city. Now enhanced with generative AI, this virtual environment simulates traffic, pedestrian flow, flood risk, energy demand, and future population changes—all in real time.
The city can "test" new roads, zoning laws, or building heights in simulation before implementing them in steel and concrete. When a major rainstorm looms, the system re-routes drainage proactively, mitigating floods with machine-calculated precision. Planning, once based on educated guesses, now runs on synthetic foresight.
Manufacturing – Self-Optimizing Factories
In the industrial heartlands of Germany, Bosch has paired digital twins with generative AI in its smart factories. Machines embedded with sensors stream continuous data. AI algorithms anticipate wear-and-tear days in advance. Production lines self-adjust in real time—shifting speeds, reallocating resources, avoiding downtime altogether.
At General Electric, AI-augmented turbines and engines simulate thousands of potential stress conditions before any part fails. In the past, a single defect could ground an aircraft for days. Now, predictive interventions keep fleets flying, saving airlines millions—and passengers, their peace of mind.
Healthcare – Personalized Engineering at the Molecular Level
Engineering used to stop at the body's edge. Now, it designs with the body. Companies like Össur and LimbForge use generative engineering to create customized prosthetics. AI scans patient anatomy, simulates gait and pressure points, and suggests designs optimized for performance, comfort, and style—all in hours.
But the most seismic shift? Drug discovery. Generative models now suggest molecular compounds never seen before. At Insilico Medicine, an AI system discovered a novel fibrosis drug candidate in under 18 months—a process that usually takes six years.
And when DeepMind's AlphaFold solved the protein folding problem—once called the "grand challenge of biology"—it collapsed a decade-long research frontier into a few keystrokes. Proteins that were once black boxes are now folded virtually, unlocking insights into diseases, drug interactions, and human biology at unprecedented speed.
Even Mathematics Is Being Engineered Differently
In 2021, Google Research and DeepMind entered the realm of pure mathematics. Their generative models, trained on mathematical theorems and proofs, began suggesting previously unexplored patterns. At the International Mathematical Olympiad, AI-assisted teams identified novel strategies to problems that had stumped competitors for years.
Mathematics, the very language of engineering, is now being extended by machines—suggesting that even our foundational tools are up for reinvention.
From Problem Solvers to System Orchestrators
Across domains—from aerospace hangars to hospital wings, from electric grids to earthquake-prone cities—a new pattern is emerging. Generative AI is not just accelerating engineering workflows. It's changing the very posture of engineering. Engineers no longer ask "What's the best solution we can compute?" They ask, "What new solutions could be possible if we let the system imagine with us?"
Traditionally, engineers were like master chess players, calculating moves within a finite board, optimizing under constraints, refining until the best feasible solution emerged. But generative AI rewrites the rules—and the board itself. It doesn't just offer faster answers. It asks better questions. It doesn't just automate tasks. It suggests new forms of reasoning.
This is the shift: from problem solvers to system orchestrators.
Engineers today stand at the helm of intelligent, dynamic systems that sense, adapt, simulate, and propose. Instead of intervening at fixed points, they now guide evolving ecosystems. They tune digital twins that learn. They co-create with agents that improvise. They balance trade-offs across scales, across time, across interdependent variables. They don't just optimize. They conduct.
Think of an engineer not as a lone architect sketching blueprints, but as a symphony conductor shaping harmony between machines, models, constraints, and goals. The system plays back—sometimes expectedly, sometimes surprisingly. And when it does, the engineer listens, adjusts, nudges, and refines the next movement.
This new identity comes with unprecedented power—and profound responsibility. Because the ripple effects of generative AI don't stop at better bridges or smarter factories. They touch labor, equity, trust, and sustainability. They shape who has access to solutions—and who doesn't. They raise hard questions about agency, accountability, and unintended consequences.
Which is why the journey ahead must not only explore what generative AI can do—but what it ought to do.
So before we begin with Trail 1 and follow the yellow brick road toward optimization, let us take a moment to map the four trails ahead—including a final one that transcends engineering's traditional boundaries. One that asks not just how engineers solve problems—but how they steward the future.
Emerging Trends and Future Directions
Charting the Future: How Generative AI Redraws the Map of Engineering
What lies ahead is not a single path but a branching, multidimensional journey—one where engineers no longer walk alone but in partnership with systems that adapt, learn, and occasionally surprise. To navigate this landscape, we offer a compass: four distinct yet interconnected trails, each illuminating how generative AI is transforming not just the tools of engineering but its tempo, terrain, and telos.
These four trails are not predictions. They are already underfoot. Based on more than 740 sources—from industry case studies and academic breakthroughs to consultant playbooks and frontline experiments—this framework distills a movement in motion. A redefinition of engineering not as a closed profession but as an evolving conversation between human intent and machine capability.
Let's walk the map together.
Trail 1: Engineering Optimization Unleashed – The End of the Trade-offs?
For decades, engineering was the art of compromise. Want lower emissions? Sacrifice speed. Want lighter weight? Increase cost. The optimization process resembled a game of Jenga—remove one block, risk destabilizing the rest. But generative AI collapses this zero-sum logic. Instead of navigating trade-offs, engineers now orchestrate harmonized multidimensional solutions—balancing constraints that were once irreconcilable.
Whether it's reducing the weight of aerospace components by 50%, or simulating urban traffic flow, energy grids, and environmental impacts in real-time, engineers are no longer constrained by what's feasible—they're guided by what's optimal across time, scale, and constraint. Optimization is no longer reactive—it's symphonic.
Trail 2: Smart Digital Twins – From Static Models to Living Mirrors
Digital twins are evolving from passive replicas to intelligent, adaptive mirrors of reality. These aren't mere visualizations. They are continuously updated, self-improving systems that anticipate, simulate, and optimize—sometimes faster than human cognition.
Picture Siemens' AI-powered factories, where digital twins predict machinery failures weeks in advance and proactively adjust workflows. Or Singapore's Virtual City, where flood risks, transit efficiency, and population growth are simulated daily. What was once static becomes fluid. The twin doesn't just reflect—it responds. And in doing so, it transforms engineering from a one-way act of creation into an ongoing dialogue with reality.
Trail 3: Generative AI as Creative Collaborator – The Rise of Engineering Intuition at Scale
In the past, engineers solved problems. Now, AI co-solves with them—surfacing patterns, proposing bold configurations, and sometimes suggesting options no human ever would. The relationship is no longer master and tool—it's more like duet and improvisation.
Think of GitHub Copilot, which reduces developer workload by up to 55%, or AlphaFold, which solved the protein-folding problem that had stumped biologists for decades. Or imagine generative AI drafting city plans that balance sunlight exposure with pedestrian flow, or proposing new materials optimized for both strength and recyclability.
Here, AI doesn't replace creativity. It scales it, turning flashes of insight into systemic possibilities. It's not just engineering faster—it's engineering deeper.
Trail 4: Sustainable Tomorrow – Engineering as Ethical Stewardship
But there is a fourth trail, less technical, more philosophical. It asks not what AI can do, but what it should do—and who gets to decide. As AI systems take on more design, decision, and operational authority, engineers step into a new role: ethical orchestrators, balancing innovation with impact, velocity with vigilance.
This trail is about embedding trust, equity, and sustainability into engineering DNA. It's about more sustainable AI, not just faster AI. It's about ensuring the benefits of automation don't deepen inequality, and that transparency isn't optional—it's foundational.
It's the trail that reminds us that engineering, at its core, is a human-centered endeavor, one that must remain grounded in the values it aims to serve.
Conclusion
Together, these four trails form a cartography of transformation. A journey from compromise to coherence, from rigidity to resilience, from tools to teammates, and from execution to ethics.
The next pages will guide you, trail by trail—beginning with Optimization Unleashed, where we explore how generative AI redefines the boundaries of what's possible when engineers no longer have to choose between performance, cost, or sustainability.
🚀 From Insight to Impact
Generative AI has redefined the logic of engineering. Now see how it unleashes optimization—where trade-offs dissolve, and multidimensional solutions emerge.
👉 Explore Trail 1: Optimization Unleashed→AI Terminology Glossary
New to AI concepts? Expand the terms below for quick definitions to help you navigate this content.