In the first three trails, we explored optimization, simulation, and problem-solving—how generative AI accelerates and amplifies what engineers can do. But Trail 4 asks a different question: What should we do?
Because the most powerful technologies do not merely reshape industries—they reshape choices. They influence how we design cities, manage energy, distribute opportunity, and define progress itself.
Generative AI opens a future of breathtaking possibility—but it does not come preloaded with wisdom. It must be guided. And that guidance, in a world of climate urgency, social fragmentation, and technological disruption, must be anchored in sustainability, equity, and trust.
Trail 4 is about that anchoring. We explore three tectonic shifts: green engineering at scale, democratization of access, and the rise of ethical AI. These are not side quests—they are the essence of engineering in the generative age.
Trend 10: Greener by Design – Engineering Sustainability as a First Principle
"Sustainability is no longer an aspiration but an equation, solved by AI's ability to transform efficiency into opportunity."
For decades, engineering progress came with a silent cost—energy-hungry systems, resource depletion, and wasteful manufacturing. Sustainability was often a retrofit, applied after the fact. Generative AI changes that. Today, sustainability is engineered upstream, embedded in the design itself—through lighter structures, more efficient systems, and intelligent prediction of lifecycle impacts.
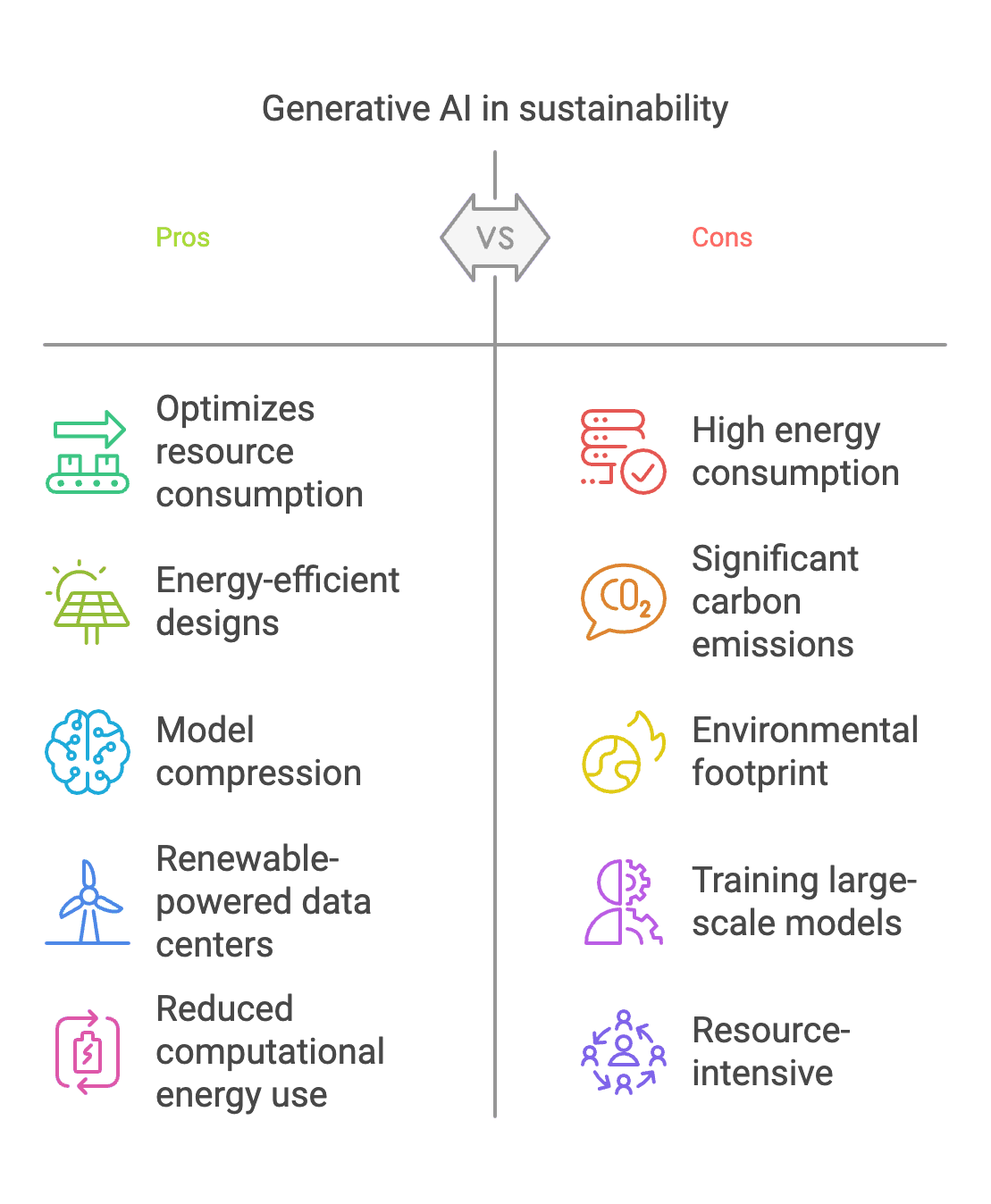
Figure 1: Generative AI and Sustainability: Balancing Innovation with Environmental Impact
AI optimizes resource consumption and energy efficiency, but its high computational demands and carbon footprint necessitate greener AI practices for long-term sustainability.
(Click image to view full size)
In data centers, DeepMind's AI slashed Google's cooling energy usage by up to 40%, optimizing airflow and thermal dynamics in real time. Across its fleet, this saved millions of kWh, cutting emissions while preserving performance.
In aerospace, Airbus used generative design to reimagine brackets and partitions, reducing component weight by 55%—without compromising safety. Each gram saved cascades into fuel savings, emissions reductions, and longer aircraft lifespans.
In energy systems, AI forecasts weather patterns to predict solar and wind output with greater than 85% accuracy, dynamically optimizing grid flows and storage, reducing reliance on fossil-fueled peaker plants.
Yet generative AI also consumes vast resources. Training a large language model emits as much CO₂ as five cars over their lifetime. The paradox is real: how do we use AI to save energy when AI itself burns so much?
The answer lies in architectural efficiency. New models—like JEPA, MoE (Mixture of Experts), and quantized transformers—deliver comparable performance with 10x fewer computations. Smart engineering is now about not just what AI can do, but how lightly it can do it.
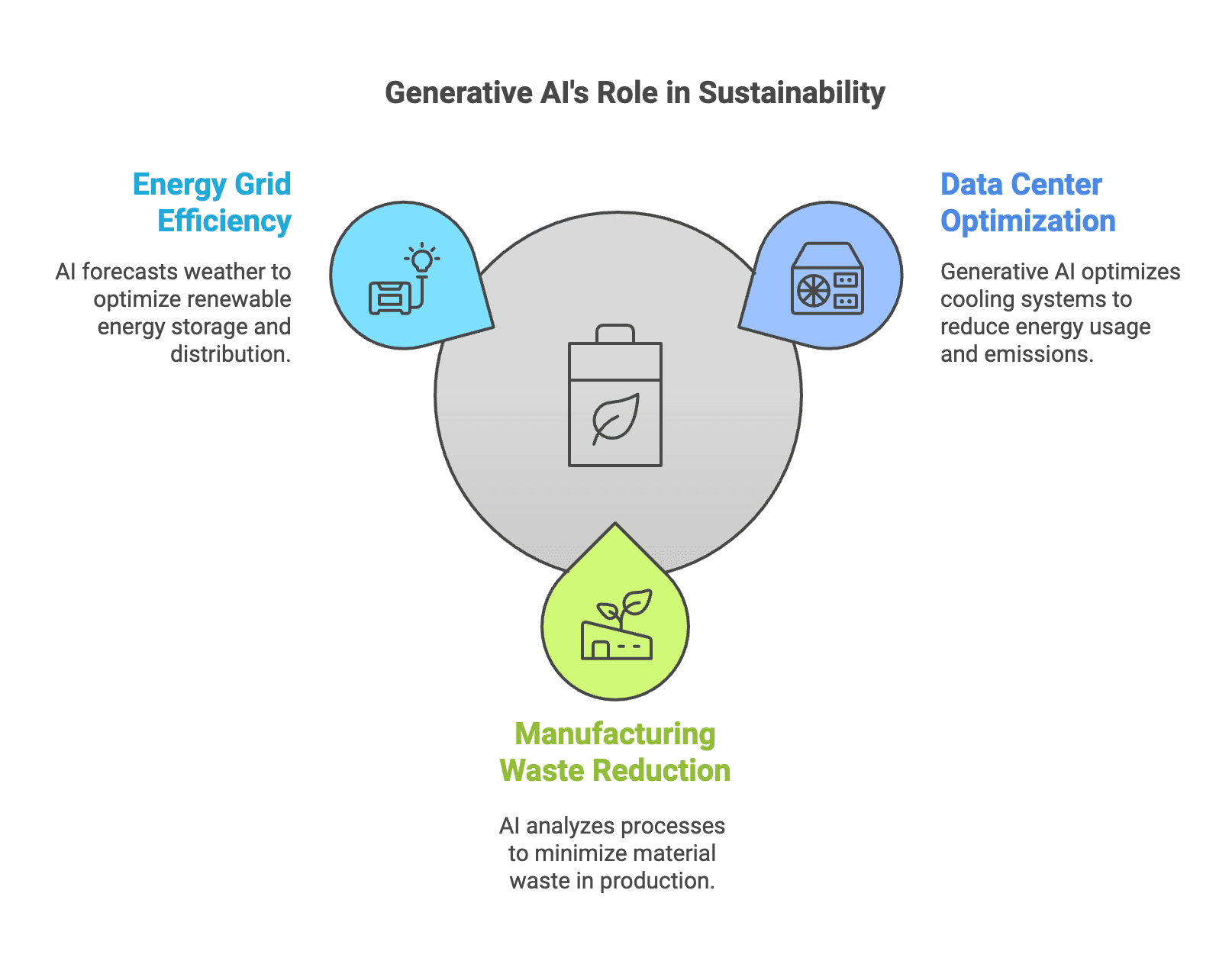
Figure 2: Generative AI's Role in Sustainability: Optimizing Energy and Reducing Waste
AI enhances sustainability by optimizing energy grids, reducing data center emissions, and minimizing manufacturing waste, balancing efficiency with environmental responsibility.
(Click image to view full size)
🔥 Cool Models, Hot Servers
AI might be brilliant—but it runs hot. Dive in to discover how today's models balance intelligence, architecture, and carbon footprints.
Trend 11: Engineering for All – Democratizing Innovation through Access
"When access to AI becomes universal, the future of innovation stops belonging to the privileged few and starts reflecting the ingenuity of the many."
For most of history, breakthrough technologies served the few—governments, corporations, elite labs. Generative AI flips that script. It lowers barriers. It turns text into software, ideas into prototypes, and data into decisions—accessible not just to engineers, but to students, small-town entrepreneurs, and community organizers.
In global education, UNESCO-supported AI labs help students in remote regions simulate experiments in physics and chemistry, improving STEM outcomes by over 50% without needing expensive equipment.
In open-source innovation, platforms like Hugging Face and GitHub Copilot empower developers in Africa, Southeast Asia, and Latin America to build locally relevant tools—from drought-resistant crop models to low-cost medical diagnostics.
In connectivity, Starlink's AI-optimized satellite constellations are reducing broadband costs by 25%, bringing fast internet to regions long ignored by traditional infrastructure.
This is not a utopia. There are risks: AI models trained predominantly on Western data risk erasing local knowledge. Infrastructure gaps still hinder equitable adoption. And without careful design, "access" can become surveillance in disguise.
But when guided ethically, AI becomes a force multiplier for human potential, redistributing opportunity the way roads, literacy, and electricity once did.
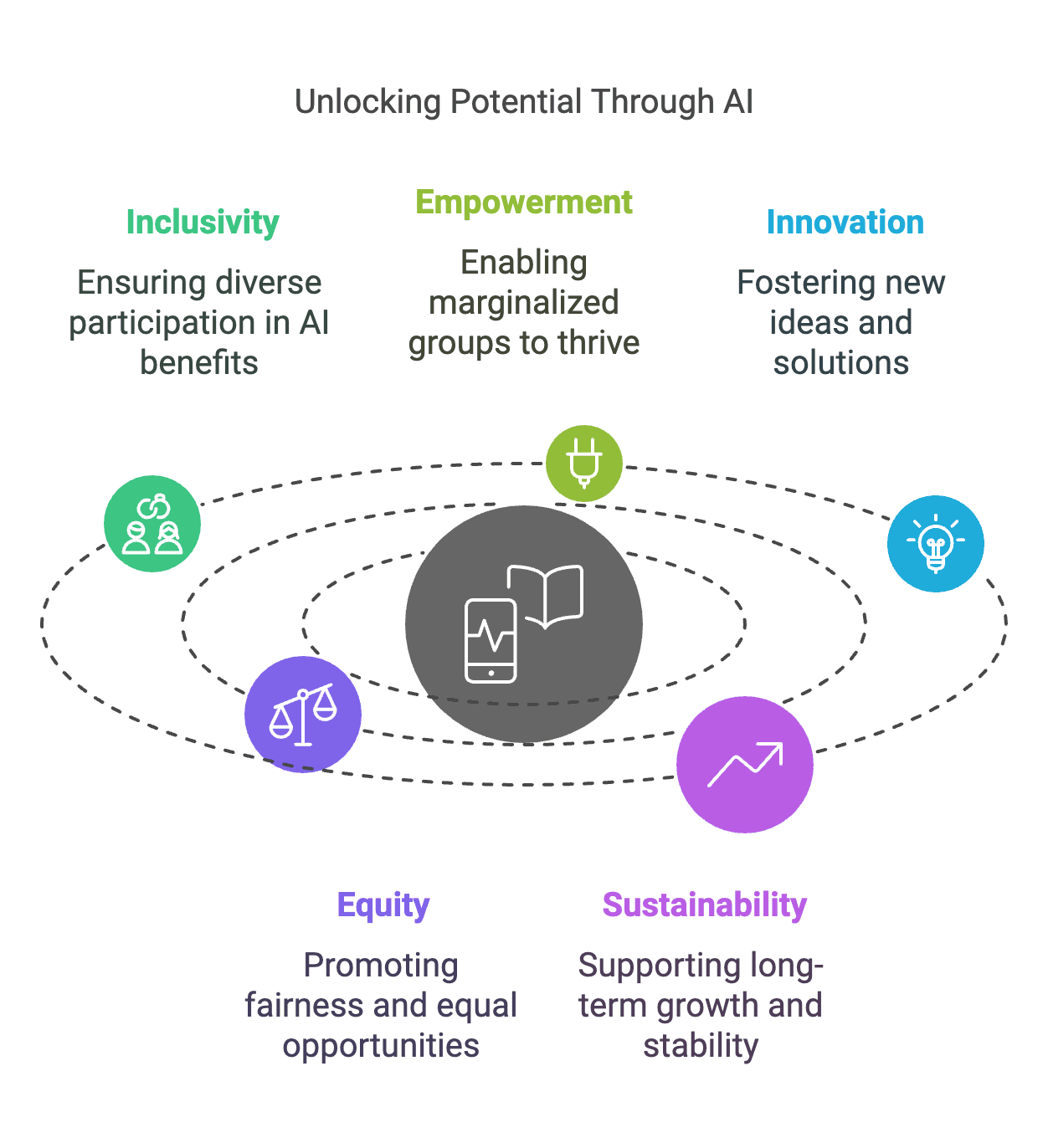
Figure 3: Unlocking Potential Through AI: Bridging Inequality and Driving Innovation
Generative AI fosters inclusivity, equity, and empowerment by democratizing access to technology, enabling innovation, and supporting sustainable growth worldwide.
(Click image to view full size)
Trend 12: Trust by Design – Building Ethical and Transparent AI Systems
"Transparency in AI isn't just a feature—it's the foundation for trust in a world where decisions are shaped by algorithms."
Generative AI systems do not merely compute. They suggest, persuade, and sometimes decide. Which makes trust not a bonus—but a requirement.
The engineer's role now includes ensuring that AI systems are fair, explainable, and accountable. That means applying not just technical checks, but ethical frameworks—international standards like ISO/IEC 23894, or the IEEE's Ethically Aligned Design.
In hiring systems, adversarial debiasing algorithms have reduced discriminatory outcomes by 35%, reshaping who gets seen, heard, and hired.
In medical diagnostics, federated learning allows hospitals to train models collaboratively—without ever exposing sensitive patient data—showcasing that privacy and progress can coexist.
In autonomous vehicles, Explainable AI (XAI) models help passengers understand why a car slowed or swerved, not just that it did. This transparency builds public trust—the true currency of next-generation systems.
And yet, the challenges remain steep. Most generative models are black boxes. They hallucinate, obscure their reasoning, and reflect the biases of their data. Engineers must dig deeper—auditing data pipelines, simulating edge cases, and embedding "ethical checkpoints" throughout development cycles.
Because when machines act on our behalf, the burden of conscience is ours.
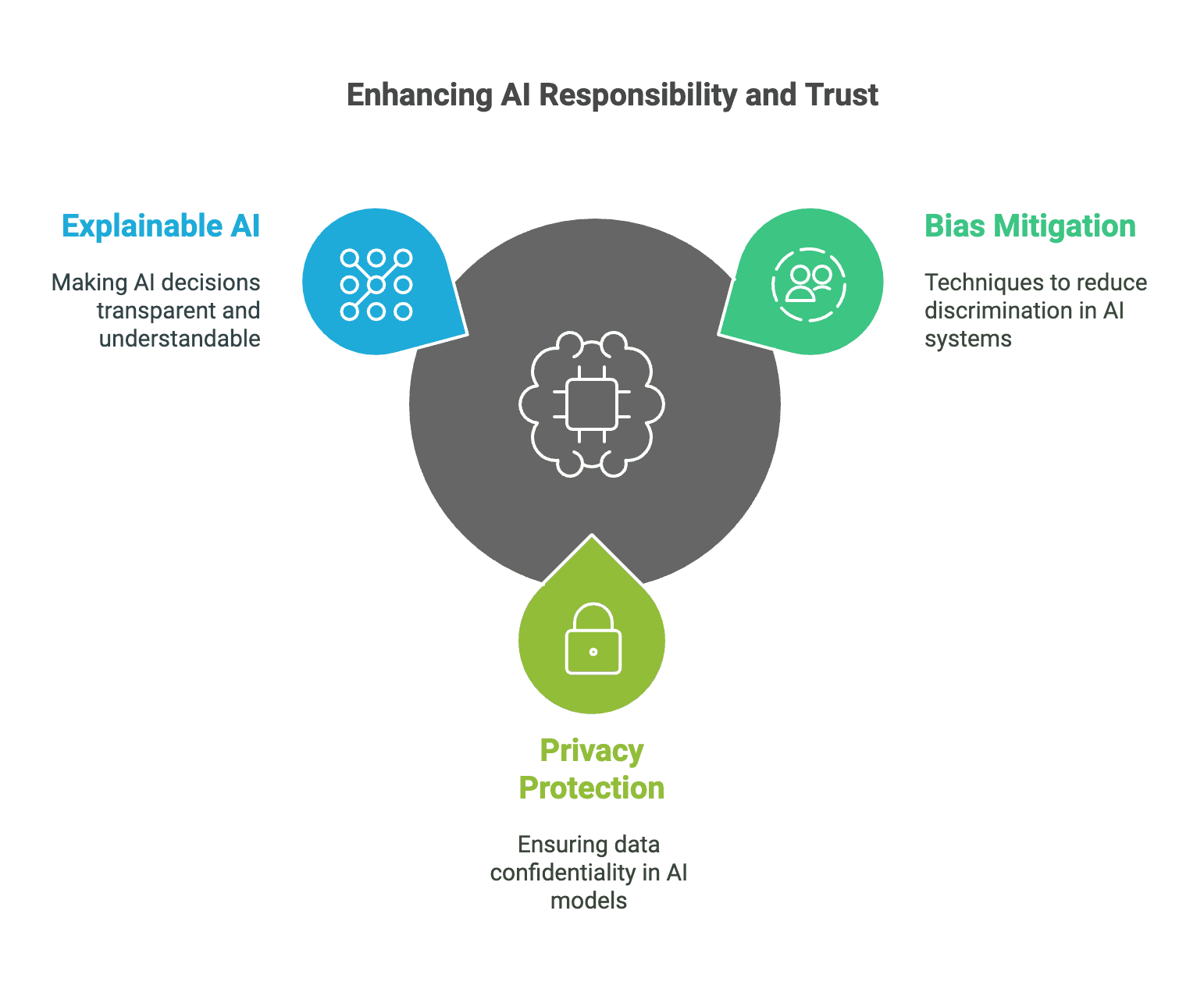
Figure 4: Building Trust in AI: Ethics, Transparency, and Fairness
For AI to be widely trusted, it must ensure explainability, bias mitigation, and privacy protection, embedding ethical principles into engineering and decision-making.
(Click image to view full size)
Conclusion
The first three trails were about capability—how AI expands what engineers can do. Trail 4 is about responsibility—what engineers ought to do.
It asks not just what is efficient, but what is right. Not just what is possible, but what is sustainable. It turns engineering from a discipline of artifacts into a philosophy of stewardship.
The French philosopher Georges Bernanos once wrote, "Hope is a risk that must be run." Generative AI offers a new kind of hope—the hope that human ingenuity, paired with machine intelligence, can meet the grand challenges of our time. But it is not guaranteed. It is a risk, and it demands that engineers run it with both ambition and humility.
In this final trail, we do not find a destination. We find a compass. One that points not just to better technology, but to better futures—futures that are just, resilient, and deeply human.
🧠 Want to dive deeper into the scaling dilemma? Read our full essay: The Cost of Scaling
🔭 Ready for What Comes Next?
You've walked the four trails. But the most important journey begins now—with the engineer.
👉 Read the Final Chapter: The Engineer's Moment→